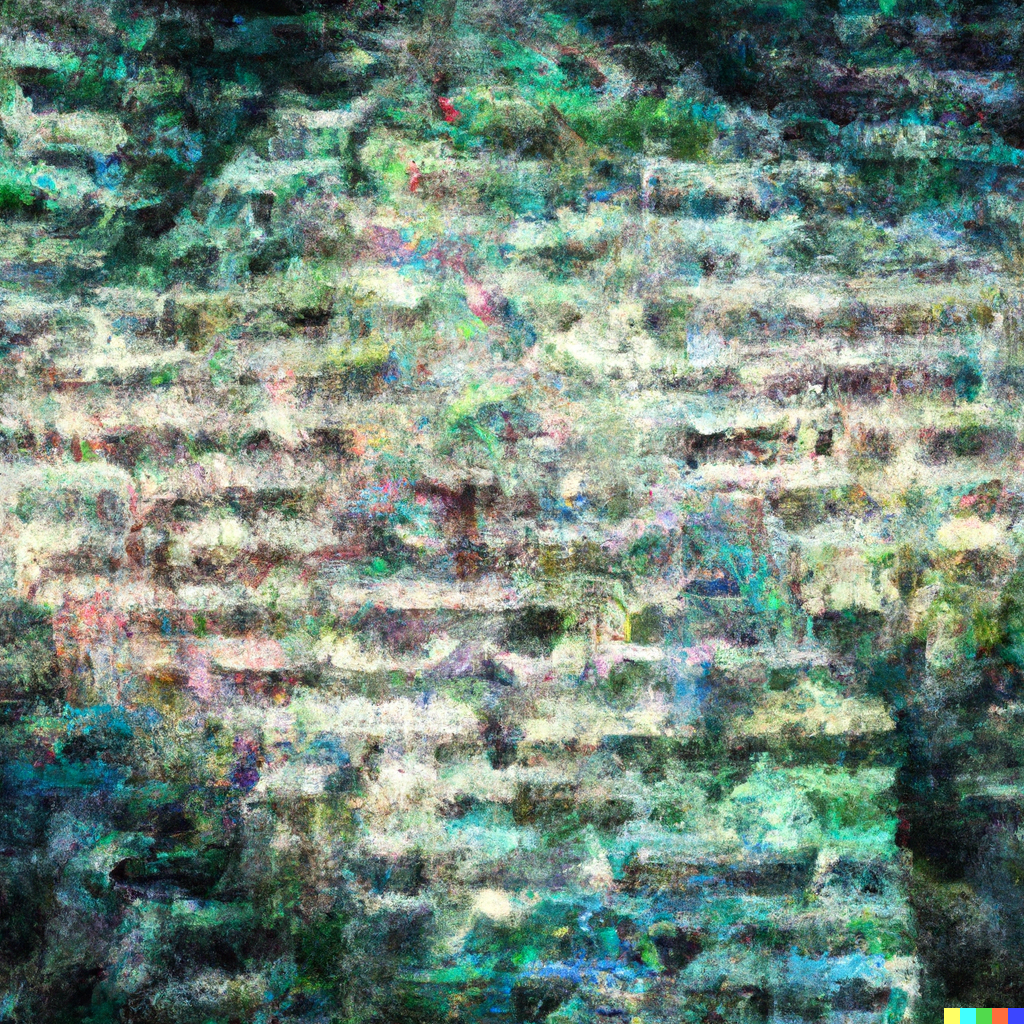
Understanding the Data Landscape in Financial Services
In the era of digitization, data has emerged as a significant asset, driving business decision-making and strategy. In the financial industry, the role of data is even more critical. Financial services institutions generate and deal with vast quantities of data daily. This data originates from multiple sources, such as banking transactions, insurance policies, customer service records, regulatory documents, investment portfolios, market data, and social media chatter, to name a few.
This data has the potential to provide rich insights into customer behavior, market trends, risk profiles, and operational efficiency. However, harnessing this value requires careful management and intelligent analysis, which becomes more complex with the data’s increasing scale and diversity.
Unstructured vs. Structured Data: Key Differences and Challenges
Data can be broadly classified into two types: structured and unstructured.
Structured data is neatly organized into tables, rows, and columns, with each piece of information having a designated place. This could include data from forms, spreadsheets, or databases, such as client names, account numbers, transaction amounts, and dates. This format is readily analyzable by traditional data processing applications.
Unstructured data, on the other hand, does not have a predefined format or organization. It could be text-heavy documents like emails, regulatory filings, social media posts, customer reviews, or more complex content types like videos, images, or audio files.
While structured data is relatively easy to store, query, and analyze, unstructured data poses significant challenges. Its heterogeneous nature makes it hard to categorize, store, and process efficiently. Moreover, due to its complex nature, traditional data analysis tools struggle to extract meaningful insights from unstructured data.
The Burgeoning Problem of Unstructured Data
The amount of unstructured data in the financial services industry is growing exponentially. It is estimated that up to 80% of business-relevant information originates from unstructured data. This could be anything from customer emails and call transcripts to market news reports and social media comments.
This explosion of unstructured data has created several challenges for financial institutions. First, storing and managing this vast quantity of data has become a logistical and economic burden. Second, the lack of effective tools and techniques to analyze unstructured data means that much of its potential value remains untapped. Finally, the regulatory implications of unstructured data, such as ensuring data privacy and compliance, add another layer of complexity to its management.
However daunting as these challenges may seem, they also represent an opportunity. Technological advancements like artificial intelligence and machine learning provide promising avenues for tackling the unstructured data problem, turning it from a burden into a strategic asset. The subsequent sections will delve into these potential solutions and guide you on how to leverage unstructured data effectively and responsibly in your financial services organization.
The Power and Potential of Unstructured Data
Unseen Value: Unstructured Data as a Strategic Asset
Unstructured data, often deemed a challenge, actually represents a vast untapped resource with significant strategic value. Hidden within the volumes of emails, documents, social media posts, and other unstructured data are valuable insights that can illuminate customer behaviors, reveal market trends, and enhance decision-making processes.
The strategic value of unstructured data lies in its richness and depth. It often contains detailed, qualitative information that structured data lacks. For instance, customer reviews can give insights into customers’ likes, dislikes, and preferences. Similarly, analysis of emails and call transcripts can reveal valuable information about customer complaints, operational inefficiencies, or emerging market trends. In other words, unstructured data is vital to understanding the ‘why’ behind the ‘what’ that structured data often provides.
Use Cases: Unstructured Data in Financial Services
Financial services firms can harness the power of unstructured data in numerous ways.
- Risk Management: Unstructured data can help identify patterns and indicators of fraudulent activities. For example, customer communication patterns or sentiment changes could hint at potential fraud or dissatisfaction.
- Customer Relationship Management (CRM): By analyzing unstructured data from customer reviews, social media, and emails, firms can gain a deeper understanding of customer needs, preferences, and sentiments, allowing them to tailor their services accordingly.
- Regulatory Compliance: Regulatory documents, often unstructured, can be analyzed for compliance purposes, ensuring that firms stay in line with the latest regulations and avoid hefty fines.
- Market Intelligence: Unstructured data from news articles, blogs, and social media can be mined for insights on market trends, competitor strategies, and customer sentiments, providing valuable intelligence for decision-making.
Barriers to Harnessing Unstructured Data
Despite its potential, several obstacles prevent financial services firms from fully harnessing the power of unstructured data.
- Data Volume and Variety: The sheer volume and diverse nature of unstructured data make it difficult to store, manage, and analyze effectively.
- Lack of Suitable Analytical Tools: Traditional data analysis tools struggle to handle unstructured data due to their complexity and diversity.
- Data Privacy and Security: Unstructured data often contains sensitive information. Ensuring the privacy and security of this data while utilizing it for insights poses a significant challenge.
- Regulatory Compliance: The need to comply with various data protection laws and regulations adds another layer of complexity to unstructured data management.
Although these barriers may seem daunting, they are not insurmountable. Moreover, technological advances, particularly in artificial intelligence and machine learning, promise to revolutionize how we manage and extract value from unstructured data. As we explore these technologies in the coming sections, we’ll uncover the strategies that financial services firms can adopt to leverage unstructured data as a powerful strategic asset.
The Regulatory Environment and Its Impact on Unstructured Data
The Regulatory Landscape in Global Financial Services
The global financial services sector is one of the most heavily regulated industries worldwide. Regulatory bodies like the U.S. Securities and Exchange Commission (SEC), the Financial Conduct Authority (FCA) in the U.K., and the European Securities and Markets Authority (ESMA), among others, maintain stringent rules and guidelines to ensure financial stability, market integrity, and consumer protection.
Key regulations impacting data management in financial services include the General Data Protection Regulation (GDPR) in the EU, the California Consumer Privacy Act (CCPA) in the U.S., and others. These laws dictate how organizations handle personal data, including its collection, processing, storage, and sharing.
Moreover, regulations specific to the financial services sector, such as the Basel III norms for banking, the MiFID II in Europe for investment services, and the Dodd-Frank Act in the U.S., have their requirements for data management, increasing the complexity of the regulatory environment.
Regulatory Challenges with Unstructured Data
The abovementioned regulations apply not just to structured data but to unstructured data. This brings a unique set of challenges:
- Data Identification and Classification: Identifying and classifying unstructured data is challenging, yet it’s a critical first step in ensuring compliance. For instance, personal information could be present in emails, documents, or social media posts, and each piece of data might be subject to different regulations.
- Data Security and Privacy: Ensuring the security and privacy of sensitive information embedded in unstructured data is a complex task. This includes managing data access, encrypting sensitive data, and effectively responding to data breaches.
- Data Retention and Deletion: Regulations often specify how long data should be retained and when it should be deleted. However, enforcing these rules on diverse unstructured data can be tricky.
Regulatory Compliance and Unstructured Data Management
Given these challenges, ensuring regulatory compliance in managing unstructured data is no small feat. However, avoiding regulatory penalties and building trust with customers and stakeholders is crucial.
Here’s where artificial intelligence and machine learning can play a vital role. Advanced data management platforms can use AI/ML to automate the identification and classification of unstructured data, ensuring it’s handled in line with applicable regulations. In addition, techniques such as natural language processing (NLP) can help parse through textual data, identify sensitive information, and even understand the context.
Furthermore, AI/ML tools can help monitor unstructured data for unusual activities, potentially averting data breaches and fraud. They can also streamline data retention and deletion processes, making it easier to comply with regulatory timelines.
The AI/ML Revolution and Its Impact on Data Management
The Evolution of AI/ML in Data Management
Artificial Intelligence (AI) and Machine Learning (ML), two of the most transformative technologies of our age, are revolutionizing data management. The ability of AI/ML to learn from data, detect patterns, and make predictions empowers businesses to derive insights from their data in ways never thought possible.
The journey of AI/ML in data management started with automating repetitive tasks and evolved to include predictive analytics, enabling businesses to anticipate future trends and events. The real game-changer, however, has been the ability of AI/ML to analyze and understand unstructured data. Techniques like natural language processing (NLP) and image recognition have enabled extracting insights from text, images, audio, and video, unearthing valuable information hidden in unstructured data.
Key AI/ML Technologies for Unstructured Data
Several AI/ML technologies are critical in dealing with unstructured data:
- Natural Language Processing (NLP): NLP enables computers to understand, interpret, and generate human language. This is invaluable for analyzing unstructured textual data, such as emails, documents, or social media posts.
- Image and Video Analysis: AI can identify objects, people, and sentiments from pictures and videos. This is useful in several applications, like fraud detection and customer experience enhancement.
- Speech Recognition: AI’s ability to transcribe and understand human speech makes it possible to analyze audio data, such as call recordings, to derive insights.
- Deep Learning: Deep learning, a subset of machine learning, uses artificial neural networks to analyze large volumes of complex data. It’s especially effective in dealing with unstructured data because it can learn and make sense of intricate patterns.
Case Studies: AI/ML Success Stories in Unstructured Data Management
Let’s look at some success stories of how AI/ML has transformed unstructured data management in the financial services industry:
- Fraud Detection: A significant bank implemented AI to monitor and analyze customer transactions, social media interactions, and call center transcripts. The system successfully identified patterns indicating potential fraud, enabling the bank to prevent fraudulent transactions and save millions.
- Customer Insights: An insurance company used NLP to analyze customer reviews and feedback. The insights gained allowed the company to understand customer pain points better, leading to improved products and services and, ultimately, higher customer satisfaction.
- Regulatory Compliance: A global investment firm leveraged AI to analyze its vast pool of emails and documents for regulatory compliance. The automated system flagged potential non-compliant communications, reducing the risk of regulatory penalties.
These examples demonstrate that despite the challenges, AI/ML technologies can unlock the potential of unstructured data, transforming it into a strategic asset. In the subsequent sections, we’ll explore how you can implement these technologies in your organization.
Designing a Roadmap for AI/ML Implementation
Building a Data-Driven Culture: The First Step
As you prepare to harness the power of AI/ML in managing unstructured data, the first and most crucial step is to create a data-driven culture within your organization. This means recognizing data as a critical business asset and making decisions based on data-derived insights rather than instinct or speculation. It involves:
- Data Literacy: Train your workforce to understand data and the insights it can provide. This doesn’t mean everyone should become a data scientist, but everyone should appreciate the value that data brings to their roles and the organization at large.
- Data Accessibility: Encourage data sharing and collaboration across teams and departments. Break down data silos to ensure everyone can access the data they need to make informed decisions.
- Data Governance: Establish policies and practices for data management. Ensure data privacy, security, and compliance with all relevant laws and regulations.
Strategy and Planning for AI/ML Adoption
Adopting AI/ML technologies requires a well-thought-out strategy and careful planning:
- Identify Use Cases: Identify areas where AI/ML can have the most significant impact, such as risk management, customer experience, or regulatory compliance.
- Invest in Technology and Skills: Evaluate and invest in AI/ML tools that fit your identified use cases. At the same time, invest in developing the skills within your organization to use these tools effectively. This might involve hiring new talent, training existing staff, or partnering with external experts.
- Implement and Iterate: Start small, perhaps with a pilot project, and gradually expand as you gain confidence and experience. Continuously learn from your experiences, measure outcomes, and refine your approach.
Overcoming Potential Challenges and Risks
The journey to AI/ML adoption is not without challenges and risks. Here’s how you can address some of the most common ones:
- Data Privacy and Security: Ensure robust data governance practices, use advanced data security tools, and educate your workforce on privacy and security protocols.
- Bias in AI/ML Models: AI/ML models learn from the data they’re fed. If this data is biased, the models will be too. Ensure your data is representative and diverse to prevent bias in your AI/ML models.
- Change Management: Introducing AI/ML may cause fear and resistance among your workforce. Manage this through transparent communication, reassurance about job security, and emphasis on the role of AI/ML as a tool to augment human capabilities rather than replace them.
AI/ML represents a significant opportunity for financial services firms to harness the power of unstructured data. You can navigate this journey successfully with a data-driven culture, a strategic approach to AI/ML adoption, and a proactive stance in overcoming challenges.
Technological Foundations of AI/ML Deployment
Essential Tech Infrastructure for AI/ML Deployment
The effective deployment of AI/ML requires a robust technological infrastructure that supports these key components:
- Data Storage and Management: Unstructured data’s volume and variety necessitate scalable and flexible storage solutions. Cloud storage, data lakes, and big data platforms are ideal.
- Computational Power: AI/ML algorithms, especially deep learning models, require high computational power. GPU-based systems and cloud computing platforms can provide the necessary resources.
- Data Security: Encryption, secure access management, and robust backup systems are essential for maintaining data privacy and security.
- AI/ML Tools: Depending on your use cases, you might need a range of AI/ML tools. These could include machine learning platforms, natural language processing libraries, and image recognition tools.
Vendor Evaluation and Selection for AI/ML Solutions
Choosing the right vendor for your AI/ML solutions is critical. Consider the following factors in your evaluation process:
- Expertise: Does the vendor have a proven track record in AI/ML, particularly in managing unstructured data? Can they provide case studies or references?
- Fit for Purpose: Does the vendor’s solution align with your identified use cases and business objectives? Do they offer the flexibility to adapt their solution to your specific needs?
- Support: Does the vendor offer adequate training and ongoing support? What is their response time for addressing issues?
- Security and Compliance: Does the vendor follow best practices in data security? Are their solutions compliant with the relevant regulations?
In-House vs. Outsourced AI/ML Development
The decision to develop AI/ML capabilities in-house or to outsource them depends on several factors:
- Skills and Resources: Do you have the in-house skills to develop and deploy AI/ML models? If not, do you have the resources to hire new talent or train your existing staff?
- Time and Cost: Developing AI/ML capabilities in-house can be time-consuming and costly. Outsourcing can provide a quicker and potentially more cost-effective solution, but it may also mean less control over the development process.
- Strategic Importance: If AI/ML is a core component of your business strategy, you might prefer to keep it in-house to maintain strategic control. On the other hand, outsourcing might be a viable option if it’s a supportive tool.
Ultimately, in-house and outsourced AI/ML development aren’t binary. For example, you might choose a hybrid approach, where you develop strategic capabilities in-house and outsource specific tasks or projects. The key is to make a decision that aligns with your business objectives, resources, and risk tolerance.
Implementing AI/ML in Unstructured Data Management
A Step-by-Step Guide to AI/ML Implementation
Implementing AI/ML in unstructured data management involves the following key steps:
- Identify the Problem: Identify the specific problems or challenges that AI/ML can help you solve. For example, these might involve risk management, regulatory compliance, customer experience, etc.
- Assemble the Team: Establish a cross-functional team comprising data scientists, IT professionals, business analysts, and other key stakeholders. This team will spearhead the AI/ML implementation process.
- Prepare the Data: Unstructured data often need preprocessing before it can be used in AI/ML models. This might involve data cleaning, formatting, and labeling.
- Develop the Model: You can now develop your AI/ML model with the data prepared. This involves selecting an appropriate algorithm, training the model on your data, and tuning it to optimize performance.
- Deploy the Model: Once the model performs satisfactorily, you can deploy it in a live environment. This might involve integrating the model with your existing IT systems and processes.
- Monitor and Improve: After deployment, continuously monitor the model’s performance and make improvements as needed. AI/ML is an iterative process, and your models will likely need tweaking and refining over time.
Case Studies: Effective AI/ML Implementation in the Financial Industry
Let’s look at a few examples of how financial firms have effectively implemented AI/ML in managing unstructured data:
- Risk Management: A large bank used AI/ML to analyze unstructured data from diverse sources, including news articles, economic reports, and social media posts. The AI/ML models successfully identified potential risk factors that were previously overlooked, enhancing the bank’s risk management capabilities.
- Customer Experience: A credit card company used NLP to analyze customer call recordings and identify common complaints and requests. The insights gained enabled the company to improve its customer service and increase customer satisfaction.
- Regulatory Compliance: An insurance company used AI/ML to monitor emails and other written communications for potential regulatory violations. The system successfully flagged non-compliant communications, helping the company avoid regulatory fines and penalties.
Monitoring and Evaluating AI/ML Effectiveness
Monitoring and evaluating your AI/ML models’ effectiveness ensures they deliver the expected benefits. Here are a few key metrics to consider:
- Accuracy: How often does the model make correct predictions? High accuracy indicates an effective model.
- Precision and Recall: Precision measures how many of the model’s positive predictions are actually correct, while recall measures how many of the actual positives the model correctly identified.
- Business Impact: Consider the model’s impact on your business objectives beyond technical metrics. For example, has it reduced risk, improved customer experience, or achieved other desired outcomes?
By diligently monitoring and evaluating your AI/ML models, you can ensure they continue providing value and meeting your business objectives. Remember that AI/ML is not a one-time initiative but an ongoing process that requires continuous improvement.
Future Trends in Unstructured Data and AI/ML
Anticipated Developments in AI/ML
As technology evolves, we can expect AI/ML to become even more integral to unstructured data management. Some anticipated developments include:
- Advances in NLP: Natural Language Processing (NLP) will continue to evolve, enabling more sophisticated analysis of text-based unstructured data. We may see AI systems capable of understanding complex semantics and even emotion in text.
- Automated Machine Learning (AutoML): AutoML is a rapidly developing field that automates the process of training machine learning models. As AutoML technologies mature, businesses can develop and deploy AI/ML models more quickly and efficiently.
- Explainable AI: As AI/ML becomes more prevalent, the demand for transparency in AI decision-making will increase. Explainable AI technologies that interpret and explain AI/ML model decisions will become more critical.
The Future of Unstructured Data in Financial Services
Unstructured data will continue to proliferate in the financial services industry. At the same time, the tools and techniques for managing this data will become more sophisticated:
- Data Fusion: Financial firms will increasingly merge unstructured data with structured data to gain deeper insights. For example, customer emails (unstructured data) might be combined with transaction data (structured data) to predict customer behavior.
- Real-time Analytics: As the technology evolves, firms can analyze unstructured data in real time. This could transform areas like risk management and customer service.
- Privacy Enhancing Technologies: As firms handle increasingly sensitive unstructured data, privacy-enhancing technologies like differential privacy and homomorphic encryption will become more critical.
Preparing for the Future: Building a Sustainable AI/ML Strategy
To stay ahead in the rapidly evolving landscape of unstructured data and AI/ML, financial firms must build a sustainable AI/ML strategy:
- Continuous Learning: AI/ML is a rapidly evolving field. Encourage a culture of continuous learning and provide opportunities for your team to update their knowledge and skills.
- Scalable Infrastructure: Build a tech infrastructure that can scale as your AI/ML initiatives grow. This might involve investing in cloud computing, big data platforms, and other scalable technologies.
- Ethical AI: As AI/ML becomes more powerful, ethical considerations will become more critical. Ensure that your AI/ML strategy includes guidelines for ethical AI use, such as fairness, transparency, and privacy.
In unstructured data and AI/ML, the only constant is change. By staying informed about the latest trends and building a flexible, future-oriented AI/ML strategy, you can navigate this change and turn it into a source of competitive advantage.
Ethical Considerations for AI/ML Use
Understanding AI Ethics and Bias
The proliferation of AI/ML applications has raised significant ethical questions. Key among these is the issue of bias:
- Algorithmic Bias: AI/ML models are only as good as the data they’re trained on. If this data is biased, the model’s predictions can also be. For instance, a loan approval model trained on historical data might disadvantage groups historically denied loans unfairly.
- Transparency and Accountability: Understanding how complex AI/ML models make their decisions can be challenging. This lack of transparency, often called the “black box” problem, can lead to unfair or unethical outcomes.
Understanding these and other ethical considerations is the first step toward responsible AI/ML use.
Creating Ethical Guidelines for AI/ML Usage
To ensure ethical AI/ML use, financial firms should create a set of guidelines that cover the following:
- Fairness: AI/ML applications should treat all individuals and groups fairly. This includes proactively addressing algorithmic bias.
- Transparency: Stakeholders should be able to understand how AI/ML models make their decisions. Where this isn’t possible, safeguards should be in place to prevent unfair or harmful outcomes.
- Privacy: AI/ML applications should respect individuals’ privacy rights, especially when handling sensitive unstructured data.
- Accountability: There should be mechanisms in place to hold individuals and groups accountable for the ethical use of AI/ML.
Companies must review these guidelines regularly to reflect new AI/ML technology developments and ethics.
Case Studies: Addressing Ethical Challenges in AI/ML
Let’s examine how some organizations have navigated ethical challenges in AI/ML:
- Bias Mitigation: A large bank discovered that its AI/ML model for loan approvals was inadvertently disadvantaging certain groups. The bank addressed this by retraining the model on a more diverse dataset and implementing regular audits to monitor for bias.
- Transparency and Accountability: A fintech company used a complex AI/ML model to predict credit risk. To enhance transparency, the company implemented an explainability layer that explains the model’s decisions.
- Privacy Protection: A financial services firm uses AI/ML to analyze customer emails for insights. To protect privacy, the firm anonymizes the emails before analysis and has strict guidelines on data use.
These examples demonstrate that while ethical challenges in AI/ML are complex, they can be effectively managed with the right approach. By prioritizing ethics and implementing strong guidelines, financial firms can harness the power of AI/ML responsibly.
Concluding Thoughts
Summary of Key Points
We’ve traversed a journey through the complex landscape of unstructured data in financial services and the role of AI/ML in managing it. Here’s a summary of the key points:
- Unstructured Data Challenge: Financial services firms are grappling with an ever-increasing volume of unstructured data. Managing this data is a significant challenge but also presents an opportunity.
- AI/ML Potential: AI/ML technologies have emerged as potent tools for managing unstructured data. These technologies can analyze unstructured data, extract insights, and support decision-making.
- Regulatory and Ethical Considerations: Navigating the regulatory landscape is challenging, especially regarding unstructured data. Ethical considerations, including fairness, transparency, and privacy, are also vital.
- Implementation Roadmap: Implementing AI/ML in unstructured data management requires a structured approach, including assembling the right team, preparing the data, developing and deploying the models, and continuous monitoring and improvement.
- Future Trends: As technology evolves, we can expect further developments in AI/ML and unstructured data management. Financial services firms should stay informed about these trends and adapt their strategies accordingly.
The Future of AI/ML and Unstructured Data in Financial Services
As we look toward the future, it’s clear that AI/ML will play an increasingly vital role in managing unstructured data in financial services. This transformation holds immense potential. By harnessing the power of AI/ML, financial firms can gain deeper insights, improve decision-making, enhance customer experience, and achieve other strategic objectives.
However, realizing this potential requires more than just technology. It requires a shift in mindset – from viewing unstructured data as a problem to be solved to seeing it as a strategic asset. It also requires a commitment to ethical and responsible AI/ML use.
With these ingredients in place, the future of AI/ML and unstructured data in financial services looks bright.