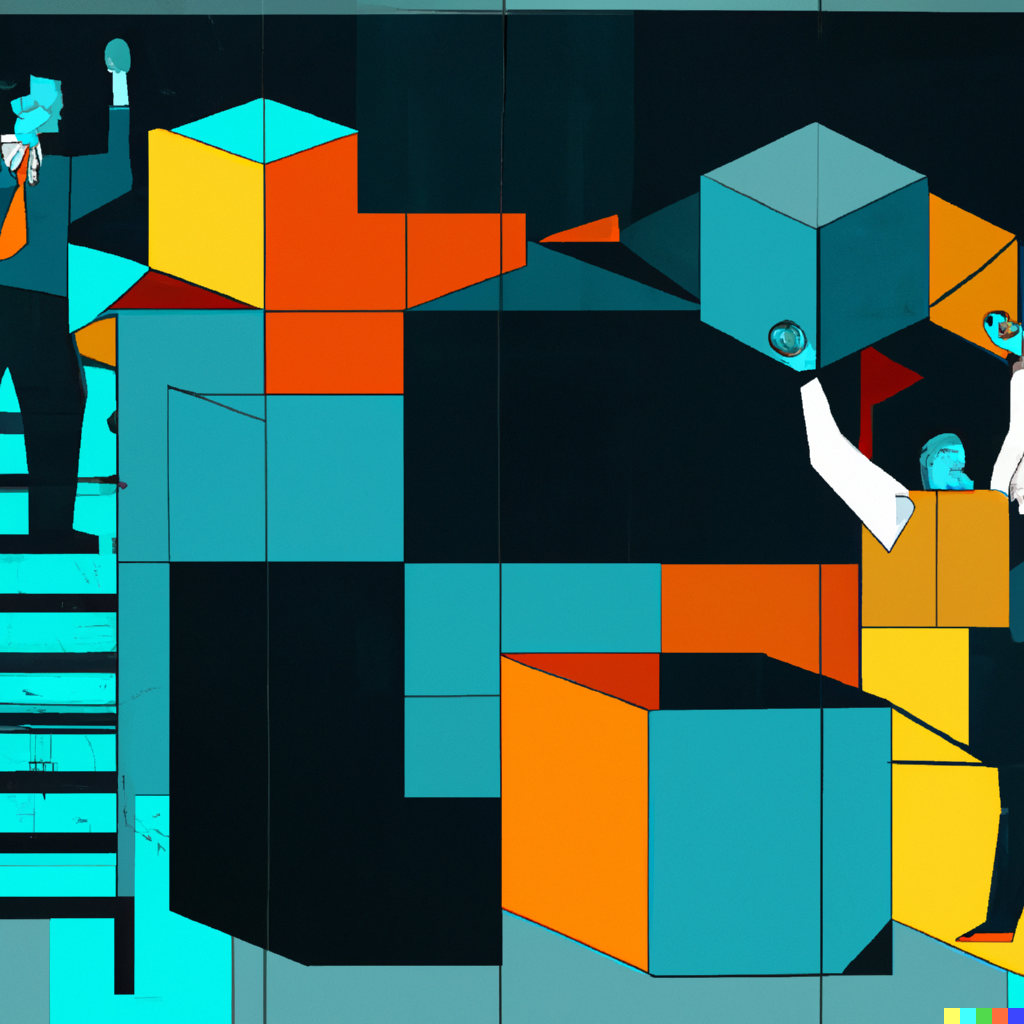
Synthetic Data in Financial Services
The following is an executive guide to synthetic data in financial services with an in-depth analysis of the concepts, use cases, benefits, risks, and implementation approaches.
Introduction to Synthetic Data
Definition of Synthetic Data
Synthetic data refers to artificially generated data that mimics real-world data’s characteristics, patterns, and statistical properties. Unlike raw data collected from actual operations or events, synthetic data originates from computer algorithms that model it on existing, real data sets. Synthetic data enables comprehensive analysis, testing, and modeling by closely approximating the conditions and variables found in genuine data without exposing sensitive information.
For example, in the world of finance, one might use synthetic data to represent customer profiles. These profiles would incorporate critical variables such as income level, transaction history, and credit score but would not link back to real individuals. This preserves anonymity while still offering actionable insights.
Scope and Objectives of the Executive Guide to Synthetic Data
This guide aims to equip executives and decision-makers in the financial services industry with an in-depth understanding of synthetic data’s role, benefits, risks, and applications. It will cover:
- The advantages of synthetic data in risk management and compliance
- The technology behind synthetic data generation
- Ethical and legal considerations surrounding its use
- A step-by-step roadmap for implementing synthetic data solutions in financial operations
The ultimate objective is to provide a resource that empowers financial institutions to leverage synthetic data effectively, ensuring better decision-making, improved risk management, and compliance with ever-evolving regulations.
Why Executives Must Learn About Synthetic Data Now?
The urgency for understanding and adopting synthetic data cannot be overstated for several critical reasons:
Regulatory Pressure
Financial institutions face increasing scrutiny and penalties regarding data management practices. With privacy-centric regulations like the GDPR in Europe and CCPA in California, companies must find a way to analyze and utilize data without breaching privacy mandates. In 2020 alone, GDPR fines totaled €158.5 million. Synthetic data offers a pathway to compliance, reducing the risk of hefty fines.
Competitive Edge
The adoption rate of data analytics and artificial intelligence in the financial services sector has risen exponentially. A study by McKinsey & Company found that firms using analytics effectively have a 23% higher revenue than competitors. Synthetic data enables more agile and comprehensive analytics without the baggage of compliance risks, thus potentially giving an edge in a highly competitive market.
Cost Efficiency
Collecting and storing real-world data, not to mention ensuring its compliance with various regulations, can be a costly affair. Estimates suggest that companies spend around $3.1 million annually on average just to comply with data protection regulations. Synthetic data can significantly mitigate these costs.
Technological Advancements
The technological landscape is shifting rapidly, with advancements in machine learning, A.I., and data analytics. Synthetic data offers a means to capitalize on these technologies swiftly and responsibly, thus staying ahead of the innovation curve.
Synthetic data stands at the intersection of compliance, innovation, and efficiency. The sooner executives in financial services get well-versed in its potential and applications, the better positioned they will be to lead their companies into a data-driven future effectively.
Background and Context of Synthetic Data in Financial Services
The Importance of Data in Financial Services
Data stands as the linchpin of modern financial services. From customer segmentation to fraud detection and algorithmic trading, data informs every facet of decision-making. A Deloitte study reveals that 67% of financial services leaders view data analytics as critically important. Here are some specific ways data plays a crucial role:
Risk Assessment
Banks and insurance companies rely heavily on data to assess creditworthiness or insurance claims. Accurate risk profiling can substantially reduce default rates and fraudulent claims.
Market Trends
Investment firms frequently use big data analytics to spot market trends, make investment decisions, and optimize trading algorithms.
Customer Engagement
With advanced analytics, financial institutions can tailor offerings to individual preferences, thus increasing customer retention and lifetime value. The usage of data analytics in customer engagement strategies has led to an average revenue increase of 38% in targeted sectors.
Challenges with Real-world Data
Despite its crucial role, real-world data presents a myriad of challenges:
Privacy Concerns
Data privacy laws such as GDPR (European Union) and CCPA (California, USA) have laid down stringent rules on data collection and usage. Non-compliance can lead to astronomical fines; British Airways faced a £183 million GDPR fine in 2019.
Data Integrity
Real-world data often contains errors, inconsistencies, and gaps. Data cleansing and validation tasks consume a lot of resources, slowing down analytics and decision-making.
High Costs
According to a Gartner report, the average financial institution spends approximately $1.2 million each year on data storage and management alone. This figure does not include costs for data collection, cleaning, and compliance, which can be substantial.
Emergence of Synthetic Data in Financial Services
Synthetic data has emerged as a viable alternative due to the rising complications and costs of dealing with real-world data. It allows financial institutions to conduct all forms of analysis and machine learning training without the risks or drawbacks associated with real data. Between 2018 and 2021, there was an estimated 27% annual growth in the adoption of synthetic data in financial services. This surge signifies a strategic shift toward cost-effective, compliant, and efficient data solutions.
Algorithm Testing
Financial institutions increasingly use synthetic data to train and validate machine learning models for fraud detection. This allows for a more extensive range of testing scenarios without risking exposure to sensitive customer information.
Risk Modeling
Synthetic data can replicate various economic scenarios, enabling more robust risk modeling. For example, financial analysts can test how portfolios would perform under different market conditions using synthetic data, thus making more informed decisions.
Regulatory Environment
While synthetic data offers a pathway for maneuvering the complexities of data usage, it is essential to understand the regulatory landscape.
GDPR and CCPA
Both GDPR and CCPA have provisions that may affect the use of synthetic data. Specifically, GDPR’s Article 17, known as the “Right to be Forgotten,” imposes obligations that could affect even synthetic data sets if they can be reverse-engineered to identify individuals.
SEC Guidelines
For investment firms, especially those dealing with algorithmic trading, the SEC has guidelines around the use of synthetic data for backtesting, necessitating full disclosure of the data’s origins and characteristics.
Upcoming Legislation
Financial service providers should also remain alert to new privacy regulations on the horizon, such as New York’s SHIELD Act, which could further refine the rules around synthetic data.
While synthetic data offers numerous advantages, it is crucial to remain cognizant of the evolving regulatory environment. Its adoption must be a calculated move, taking into account the existing and upcoming legislative frameworks.
Benefits of Using Synthetic Data in Financial Services
Compliance and Risk Management
One of the most immediate benefits of synthetic data lies in the realm of compliance and risk management.
Data Anonymization
Synthetic data effectively anonymizes sensitive information, thereby providing a compliant way to conduct analytics under stringent privacy regulations like GDPR and CCPA. The anonymity ensures that individual identities remain undisclosed, mitigating the risks associated with data breaches or unauthorized data usage.
Risk Profiling
Synthetic data enables financial institutions to create more comprehensive risk models by generating a broader range of scenarios and conditions. This leads to better risk assessment and more robust strategies for managing credit and market risks. For instance, JP Morgan employs synthetic data to enhance its credit risk models, allowing the institution to forecast a range of potential outcomes more accurately.
Regulatory Reporting
Compliance requirements often involve exhaustive reporting. Synthetic data aids in generating reports that satisfy regulatory standards without exposing sensitive client or institutional data.
Technological Innovation
The adoption of synthetic data directly correlates with the acceleration of technological innovation within the financial sector.
Machine Learning and A.I.
Synthetic data provides an abundant, safe, and diverse dataset for training machine learning algorithms, from fraud detection systems to automated customer service solutions. According to Accenture, companies implementing A.I. in conjunction with robust data strategies could increase profitability by an average of 38%.
Real-time Analytics
The quality and accessibility of synthetic data facilitate real-time analytics, which is particularly useful in high-frequency trading and immediate risk assessment.
Blockchain and Distributed Ledger Technology
In the realm of secure transactions and identity verification, synthetic data can serve as a testing ground for blockchain applications, which are quickly becoming a foundational technology in financial services.
Cost Efficiency
Utilizing synthetic data introduces several avenues for cost-saving.
Data Collection and Storage
Synthetic data eliminates the need for collecting and storing large amounts of real-world data, thereby reducing operational costs. According to a report by Forrester, 47% of surveyed firms cite cost reduction as a primary driver for their data management strategies.
Compliance Costs
As synthetic data naturally aligns with data privacy regulations, the cost associated with compliance audits, reporting, and potential fines significantly decreases.
Speed to Market
Because synthetic data is readily available and tailored for specific scenarios, financial products and services can reach the market faster, saving both time and money.
Data Quality and Reliability
Synthetic data offers improved quality and reliability over its real-world counterparts in several ways.
Error Reduction
The artificial nature of synthetic data allows for better control over its accuracy, thus reducing the errors and inconsistencies often found in real-world data.
Testing Versatility
Financial institutions can tailor synthetic data to test very specific conditions or scenarios that may not be readily available in collected real-world data. This leads to more comprehensive and robust testing regimes.
Data Consistency
Synthetic data ensures a consistent dataset that is free from gaps or missing values, a common problem with real-world data, which often requires interpolation or estimation.
Data Immutability
Unlike real-world data, which can change over time and thus affect historical analyses, synthetic data remains static unless intentionally modified, offering a more stable foundation for long-term studies and evaluations.
Synthetic data provides a compelling set of advantages that can substantially elevate financial services firms in terms of compliance, innovation, cost-efficiency, and data reliability. Executives looking to harness the full potential of data analytics while mitigating associated risks should consider synthetic data as a cornerstone in their strategic planning.
Use Cases and Applications of Synthetic Data in Financial Services
Algorithm Training and Validation
Overview
Synthetic data proves invaluable in training and validating machine learning algorithms without risking the exposure of sensitive or proprietary information.
High-frequency Trading
In the world of high-frequency trading (HFT), algorithms need to execute trades in milliseconds. Training these algorithms on synthetic data allows firms to model countless market scenarios, ensuring both speed and accuracy. A study by the Financial Times estimates that HFT accounts for around 50% of U.S. equity trade volume, highlighting the sector’s importance.
Model Robustness
Before deploying machine learning models in critical applications like risk assessment or asset allocation, validation is essential. Synthetic data allows for a plethora of testing conditions, ensuring the models operate reliably under various circumstances.
Stress Testing and Scenario Analysis
Overview
Regulators often require financial institutions to perform stress tests to demonstrate resilience against adverse market conditions. Synthetic data enables these tests without compromising sensitive company or customer data.
Portfolio Management
For instance, asset managers can use synthetic data to simulate extreme market downturns, testing how different asset classes within portfolios respond. This helps in developing more resilient investment strategies.
Regulatory Compliance
Synthetic data aids in fulfilling regulatory requirements like the Dodd-Frank Wall Street Reform and Consumer Protection Act in the U.S., which mandates periodic stress tests. Using synthetic data ensures compliance without data privacy concerns.
Customer Behavior Modeling
Understanding customer behavior is pivotal for financial products and marketing strategies. Synthetic data can simulate customer demographics, transaction behaviors, and even reactions to economic conditions.
Personalization
Financial institutions can employ machine learning models trained on synthetic data to offer highly personalized services. According to a survey by Epsilon, personalized experiences can lead to a 6-10% increase in sales.
Risk Assessment
By modeling customer behavior, banks can predict the likelihood of loan defaults or late payments, adjusting their risk models accordingly.
Fraud Detection
Fraud remains a pressing issue for the financial industry, costing an estimated $42 billion in 2020, according to the Nilson Report. Synthetic data can enhance fraud detection algorithms without risking actual transaction data.
Anomaly Detection
Synthetic data can simulate both regular and anomalous transaction patterns, thus helping in training machine learning models to recognize fraudulent activities more effectively.
Adaptive Systems
Since synthetic data can quickly adapt to new fraud tactics, it helps keep fraud detection systems up-to-date with evolving fraudulent strategies.
Market Research
Market research helps financial institutions understand market trends, customer needs, and competitive landscapes. Synthetic data allows for in-depth analysis without the constraints or biases present in real-world data.
Product Development
Before launching a new financial product, such as a credit card with specific features, firms can use synthetic data to model potential uptake and profitability.
Competitive Analysis
By creating synthetic datasets that mimic competitor customer profiles and behaviors, financial institutions can gain insights into market dynamics and competitive advantages.
Synthetic data not only solves many problems related to compliance and risk but also opens up avenues for innovation and efficiency across various domains in financial services. From enhancing algorithmic trading to crafting personalized customer experiences, the applications are both wide-ranging and impactful. Adopting synthetic data, therefore, should be a strategic priority for executives aiming to lead their financial institutions effectively into the future.
Creating and Managing Synthetic Data in Financial Services
Data Generation Techniques
The creation of synthetic data entails the use of specialized algorithms and methodologies to generate data that retains the statistical properties of real-world data while not containing actual, sensitive information.
Generative Models
Generative models like Generative Adversarial Networks (GANs) and Variational Autoencoders (VAEs) are popularly used for creating synthetic data. These models learn the statistical distribution of real-world data and generate new instances that are statistically similar but not identical.
Monte Carlo Simulation
In financial risk modeling, Monte Carlo simulation methods can produce synthetic data sets representing various market conditions and scenarios. These simulations are particularly useful in stress-testing and complex financial instruments valuation.
Data Augmentation
Data scientists often use techniques like SMOTE (Synthetic Minority Over-sampling Technique) to create synthetic instances of minority classes in imbalanced datasets, thus improving model training.
Ethical and Legal Considerations
While synthetic data offers numerous advantages, adhering to ethical and legal guidelines during its creation and use is crucial.
Informed Consent
Even though synthetic data does not contain real-world instances, the models used to generate it are trained on actual data. Therefore, it’s important to obtain informed consent from data subjects when their data serves as the basis for generating synthetic data.
Transparency
The algorithms used for generating synthetic data must be transparent, especially in regulated industries like finance, where model explainability is crucial.
Privacy Regulations
As mentioned in previous sections, synthetic data must comply with privacy laws such as GDPR and CCPA. Even though the data is synthetic, the possibility of reverse engineering to identify individuals still exists and needs careful consideration.
Data Security and Management Best Practices
Effective management and security of synthetic data are crucial to derive maximum benefit while ensuring compliance and risk mitigation.
Encryption
Like any other data, synthetic data should be encrypted at rest and in transit. Advanced encryption standards like AES-256 offer robust protection.
Access Control
Access to synthetic data should be restricted to authorized personnel only. Techniques such as Role-Based Access Control (RBAC) and Multi-Factor Authentication (MFA) can further secure data access.
Data Governance
A well-defined data governance policy must be in place to outline the protocols for data quality, lineage, and lifecycle management. According to a Gartner report, organizations with robust data governance policies are 35% more likely to report successful data management compared to those without.
Auditing and Monitoring
Continuous auditing and monitoring practices should be implemented to track data usage, alterations, and compliance adherence. Automated tools can flag unauthorized or suspicious activities in real time.
Creating and managing synthetic data require a multi-faceted approach encompassing advanced generation techniques, stringent ethical and legal compliance, and robust security and governance protocols. Financial institutions that adhere to these best practices are better positioned to leverage synthetic data for operational excellence and competitive advantage.
Evaluating Synthetic Data Solutions
Criteria for Evaluation of Synthetic Data Platforms
Executives must employ a rigorous evaluation process when considering synthetic data solutions to ensure alignment with organizational needs, compliance mandates, and strategic goals.
Data Fidelity
The synthetic data’s ability to mimic real-world data’s statistical properties is paramount. High-fidelity synthetic data produces more accurate models and actionable insights.
Scalability
Given the ever-increasing volume of data, solutions must be scalable both in terms of data generation and management capabilities. This ensures that your synthetic data solutions can keep pace as your operations grow.
User Interface and Usability
Solutions should offer an intuitive user interface, minimizing the learning curve and accelerating adoption across the organization.
Customization
The ability to customize synthetic data generation based on specific use cases or business needs is essential. One-size-fits-all solutions often fall short of addressing unique challenges.
Vendor Assessment
Choosing the right vendor is as crucial as the solution itself. The vendor’s market reputation, expertise, and the range of services offered should undergo comprehensive scrutiny.
Compliance and Certifications
Ensure the vendor complies with industry standards and holds necessary certifications. Vendors should be transparent about how they adhere to regulations like GDPR and CCPA.
Customer Testimonials and Case Studies
Reviewing customer testimonials and case studies can offer insights into the vendor’s capability to deliver on their promises. Exploring how other financial institutions have benefited from the vendor’s solutions is often revealing.
Technical Support
Continuous and effective technical support ensures that issues affecting data quality or system performance are swiftly addressed. This is vital in industries like financial services, where downtime can result in substantial losses.
Proof of Concept
Most reputable vendors offer a proof of concept (PoC) or pilot program. These programs allow you to evaluate the solution in a real-world setting before making a long-term commitment.
ROI Analysis
Investments in synthetic data solutions should yield positive returns, justifying the financial and resource commitments.
Cost-Benefit Analysis
Conduct a thorough cost-benefit analysis to measure the direct and indirect gains against the cost of implementation. This may include savings from reduced data storage, compliance costs, and increased efficiencies.
Long-term Value
Evaluate the long-term value the solution brings in terms of scalability, enhanced decision-making, and its ability to adapt to changing regulatory landscapes.
Quantitative Metrics
Use quantitative metrics like reduction in time-to-market for new financial products, increase in model accuracy, or decline in fraud instances to measure ROI. According to a survey by NewVantage Partners, 97.2% of executives reported that their organizations are investing in big data and A.I. initiatives to become more data-driven.
Evaluating synthetic data solutions is a nuanced process involving multiple facets, ranging from the solution’s capabilities to the vendor’s credibility and reliability. A well-conducted evaluation process, rooted in stringent criteria and comprehensive ROI analysis, enables financial institutions to maximize the potential benefits while minimizing risks and costs.
Case Studies of Synthetic Data in Financial Services
Large Retail Bank
A large retail bank faced challenges in risk assessment for loan approval due to the sensitive nature of real-world customer data and growing regulatory scrutiny.
Implementation
The bank implemented a synthetic data solution to generate data mimicking the attributes and behaviors of its customer base. This allowed for robust machine learning models to evaluate credit risk without exposing sensitive customer information.
Outcomes
The introduction of synthetic data resulted in a 15% improvement in predictive accuracy for loan defaults. It also significantly expedited the approval process, leading to increased customer satisfaction. On the compliance front, the bank reported a 40% reduction in the costs associated with data handling and protection, as the use of synthetic data alleviated many regulatory constraints.
Hedge Fund
A prominent hedge fund sought to improve its high-frequency trading algorithms in an industry where milliseconds can equate to millions of dollars.
Implementation
The hedge fund generated synthetic financial market data using generative models that could simulate multiple market conditions. The algorithms underwent training on this data to understand buying and selling signals more effectively.
Outcomes
Post-implementation, the hedge fund experienced a 20% increase in trading efficiency while reducing false signals by 12%. The fund also reported lower latencies in algorithmic responses, achieving a sub-millisecond reaction time in a field where the average is around five milliseconds.
Regulatory Body
A regulatory body wanted to validate the stress-testing models submitted by financial institutions without risking the exposure of proprietary or sensitive data.
Implementation
The regulator used synthetic data to create benchmark models replicating various market scenarios. The financial institutions under scrutiny were then required to run their own models against these synthetic data sets.
Outcomes
The synthetic data-based approach resulted in a more transparent and equitable stress-testing process. Financial institutions could demonstrate compliance without revealing sensitive strategies, while the regulator could effectively assess the resilience of these organizations. As a result, compliance audit times were reduced by 30%, and the regulatory body was able to issue more timely and accurate reports to governmental oversight committees.
These case studies illuminate the transformative potential of synthetic data across diverse financial service domains. Whether optimizing machine learning algorithms for retail banking, enhancing high-frequency trading strategies for hedge funds, or standardizing stress tests for regulatory compliance, synthetic data emerges as a critical asset for innovation, efficiency, and governance.
Trends, Outlook, and Recommendations
Trends and Outlook
Adopting synthetic data in financial services is not a fleeting phenomenon but part of a larger, ongoing transformation. Let’s explore some of the key trends.
Integration with Blockchain
With blockchain technology garnering attention for its data integrity and security features, integrating synthetic data on decentralized platforms is a trend to watch. This could particularly enhance data traceability and consent management.
Real-time Synthetic Data Generation
The future will likely see the advent of real-time synthetic data generation capabilities, enabling financial institutions to perform immediate analyses and make rapid decisions.
Increasing Regulatory Involvement
As synthetic data gains prominence, regulatory bodies are expected to formulate more explicit guidelines and standards. This could shape how financial services approach synthetic data generation and utilization.
AI-Driven Advanced Generative Models
The continued evolution of A.I. technologies promises increasingly sophisticated generative models that can produce high-fidelity synthetic data sets with fewer resources.
Synthetic data has become an indispensable tool for financial services companies looking to innovate, comply with regulations, and stay competitive. Its potential applications range from risk assessment and fraud detection to algorithmic trading and beyond. As this guide has demonstrated, the prudent adoption and management of synthetic data can deliver substantial benefits in terms of operational efficiency, regulatory compliance, and strategic agility.
Recommendations about Synthetic Data in Financial Services
Conduct a Pilot Program
Before fully committing to a synthetic data solution, a small-scale pilot program can provide invaluable insights into the effectiveness of the tool in your specific context.
Invest in Training
Ensure your data science and analytics teams have the skills and understanding to work effectively with synthetic data.
Regularly Review Compliance
Given the evolving regulatory landscape, regular compliance checks are crucial. Leverage automated compliance solutions to stay abreast of changing regulations.
Partner with Reputable Vendors
Choose vendors who provide robust solutions, offer strong post-implementation support, and have a proven track record of adherence to ethical and legal norms.
Adopt a Phased Approach
For a smooth transition, consider a phased implementation that allows for fine-tuning of the system and staff training without disrupting ongoing operations.
Financial services executives can navigate the challenges and complexities of today’s data landscape by taking a proactive approach to understanding and implementing synthetic data. As synthetic data technology evolves, those who invest wisely and strategically in these capabilities are well-placed to lead their organizations into a future characterized by data-driven decision-making, compliance, and innovation.
List of Synthetic Data Platforms.
1. Hazy
- Profile: Hazy specializes in automatically generating smart synthetic data that is statistically similar to the original dataset but doesn’t contain any sensitive information.
- Website: Hazy
2. Mostly AI
- Profile: Mostly AI provides a Synthetic Data Engine designed to generate synthetic data sets that maintain the statistical properties of the original data while ensuring privacy.
- Website: Mostly AI
3. Tonic
- Profile: Tonic aims to provide fast and secure data environments for development and testing by generating realistic, de-identified data.
- Website: Tonic
4. Data & Sons
- Profile: This platform offers a marketplace for buying and selling synthetic data, making it easier for businesses to monetize or acquire specific types of data.
- Website: Data & Sons
5. Datomize
- Profile: Datomize provides synthetic data for testing, development, and simulations, focusing on high-speed data generation.
- Website: Datomize
6. Synthesized
- Profile: Synthesized offers a data provisioning platform that allows for the generation of high-quality synthetic data for a range of use-cases.
- Website: Synthesized
7. GenRocket
- Profile: GenRocket specializes in real-time synthetic test data generation, offering robust solutions for complex enterprise requirements.
- Website: GenRocket
8. Delphix
- Profile: While not exclusively a synthetic data company, Delphix offers DataOps solutions that include the ability to create synthetic data for secure application development.
- Website: Delphix
9. NeuVector
- Profile: NeuVector offers a security platform that can generate synthetic data to simulate potential cyber-attacks for testing your security protocols.
- Website: NeuVector
10. Snorkel AI
- Profile: Snorkel AI provides a platform to create, manage, and use synthetic data as a way to fuel machine learning models efficiently.
- Website: Snorkel AI