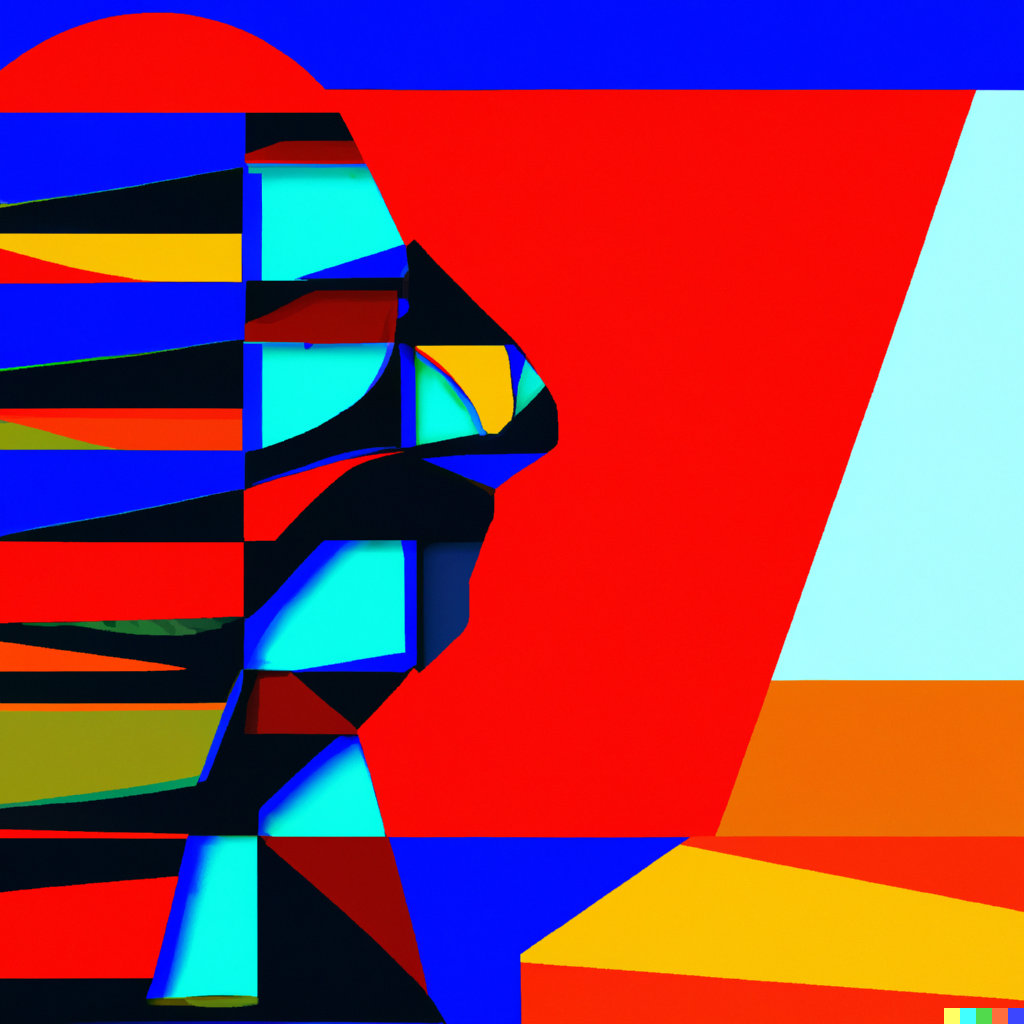
Banks deal with numerous risks daily, but credit risk is perhaps the most significant. Simply put, credit risk is the potential that a borrower will default on a loan, leading to financial loss for the lender. Managing credit risk effectively is critical for maintaining the bank’s financial health and ensuring trust among its customers and investors. However, traditional credit risk management can be fraught with challenges, such as antiquated systems, data silos, cumbersome processes, and reliance on limited, often outdated, risk models. This can create a blind spot in a bank’s risk management approach, leaving it vulnerable to unforeseen credit events.
From Compliance Necessity to Business Agility Tool: A New Perspective on Credit Risk Management
Historically, credit risk management has often been seen as a regulatory checkbox necessary for compliance. While meeting regulatory requirements remains essential, this view limits the potential value that effective credit risk management can bring. Instead, forward-thinking banks are beginning to understand credit risk management as a strategic tool for business agility and profitability.
Banks can control losses, optimize capital allocation, and identify profitable lending opportunities by actively managing credit risk. This approach transforms credit risk management from a mere defensive strategy to an offensive one, providing a competitive advantage in today’s volatile banking environment.
The Emergence of Analytics, Machine Learning, and A.I. in Banking
The advent of advanced analytics, machine learning, and artificial intelligence (A.I.) is now set to revolutionize credit risk management. These technologies offer powerful tools for analyzing vast amounts of data, predicting potential credit events, and even automating decision-making processes, thus overcoming many limitations of traditional credit risk management.
With these technologies, banks can significantly improve their ability to predict and manage credit risk, leading to lower losses, higher profitability, and greater business agility. However, harnessing these new tools requires a deep understanding of their potential and challenges and a shift in mindset among risk management professionals and executives.
This whitepaper aims to provide a comprehensive overview of how analytics, machine learning, and A.I. are reshaping credit risk management. Through this, we hope to empower you to leverage these technologies effectively, transforming credit risk management in your organization from a regulatory necessity to a strategic asset.
Understanding the Current State of Credit Risk Management
Deconstructing Credit Risk Management – Definition, Components of Credit Risk, Factors that influence credit risk for Borrowers
Credit risk management refers to the procedures, practices, and systems a financial institution uses to identify, assess, measure, and mitigate the risk of loss due to borrowers’ non-repayments. It’s important to appreciate that credit risk comprises several components. One is default risk — the risk that borrowers will not meet their repayment obligations. Another is concentration risk, which arises when a disproportionate amount of the bank’s portfolio is concentrated in a particular sector or type of loan. Finally, there’s also a country risk, where geopolitical or economic instability can increase the likelihood of defaults.
Several factors influence a borrower’s credit risk. These include their credit history, income stability, current debt level, and economic conditions. For example, during the 2008 financial crisis, many homeowners defaulted on their mortgage payments due to declining house prices and increased unemployment rates.
Deep Dive into Credit Risk Management: Concepts, Scope, and Importance
The scope of credit risk management extends beyond merely assessing a borrower’s ability to repay a loan. It also involves managing the institution’s overall exposure to credit risk, which includes setting risk appetite and limits, managing risk concentrations, and ensuring compliance with regulatory requirements.
Credit risk management is crucial to the financial stability and success of banks. With robust credit risk management, banks can mitigate losses, optimize capital allocation, improve profitability, and maintain investor confidence. For example, JP Morgan Chase, which had a solid credit risk management system, navigated the 2008 financial crisis better than many other banks.
Limitations and Challenges of Traditional Credit Risk Management Systems
Traditional credit risk management systems, often heavily reliant on manual processes and outdated technology, face several limitations. First, these systems typically rely on backward-looking data, such as a borrower’s past credit history, which may not accurately predict future behavior. Second, legacy systems often operate in silos, limiting the ability to gain a holistic view of a bank’s credit risk exposure.
Further, these systems can be slow and inefficient, requiring significant resources to manage and update. They also struggle to incorporate more complex risk factors, such as changes in the broader economy or industry-specific trends. For example, many banks were caught off-guard by the scale of defaults in the subprime mortgage sector leading up to the 2008 financial crisis, revealing a significant shortcoming in traditional risk management systems.
The Impact of Ineffective Credit Risk Management: Financial and Regulatory Consequences
The consequences of ineffective credit risk management can be severe. Financially, high default rates can lead to significant losses for banks. For instance, during the 2008 financial crisis, Citigroup, which had considerable exposure to subprime mortgages, suffered heavy losses of over $27 billion in 2008 alone.
From a regulatory perspective, banks that fail to manage credit risk effectively can face sanctions, including fines and restrictions on business activities. Severe regulatory penalties can sometimes threaten a bank’s operating license. Additionally, ineffective credit risk management can damage a bank’s reputation, losing customer trust and potential business. A precise instance is the case of Wells Fargo, which faced severe reputational damage and regulatory penalties due to poor risk management practices in the mid-2010s.
In sum, while complex, credit risk management is crucial for banks’ survival and success. Despite its challenges, the advent of advanced technologies promises to transform this vital function, providing opportunities for banks to manage credit risk more effectively and strategically.
The Power of Data in Credit Risk Management
The Role of Historical and Forecast Data in Credit Risk Management
Data is the lifeblood of credit risk management. Historical data, such as a borrower’s past credit behavior, payment history, and financial transactions, is often used to evaluate their creditworthiness. Banks use credit scoring models that draw on this data to predict the likelihood of default. For example, a borrower with a history of late payments is generally considered a higher risk.
However, historical data alone may not be sufficient to predict future credit behavior, particularly in rapid change. This is where forecast data comes in. By incorporating macroeconomic indicators, industry trends, and even alternative data such as social media behavior or utility payments, banks can enhance their credit risk models, improving their ability to forecast risk.
In 2012, the Federal Reserve introduced stress testing for large banks, requiring them to demonstrate that they can withstand severe economic downturns. This has encouraged banks to incorporate forecast data into their risk models. For instance, Goldman Sachs uses machine learning models to forecast economic scenarios and evaluate how these scenarios might impact its credit portfolio.
Overcoming Data Silos: Integrated Systems for Effective Risk Analysis
Data silos present a significant challenge for effective credit risk management. In many banks, data is stored in separate systems or databases that don’t communicate with each other. This fragmented approach can lead to a disjointed view of a borrower’s credit risk and hinder the bank’s ability to manage its overall risk exposure.
Integrated data systems can help overcome this challenge by combining data from across the bank, enabling a more holistic view of credit risk. These systems can consolidate data from different business lines, geographies, and types of loans, providing a comprehensive view of the bank’s credit risk profile.
For example, in response to the financial crisis 2008, many banks have invested in integrated risk data systems. JPMorgan Chase, for instance, reportedly spent over $500 million to create a central data repository that consolidates data from across its various business lines, enhancing its ability to manage risk.
Case Studies: Success Stories of Banks Harnessing Data for Risk Management
Several banks have successfully leveraged data to enhance their credit risk management.
One example is BBVA, a Spanish bank that uses big data analytics to improve its credit risk assessment. BBVA has developed more accurate and predictive credit scoring models by combining traditional financial data with alternative data sources. As a result, the bank has reportedly reduced its default rates and improved its lending efficiency.
Another example is Barclays Africa, which implemented a data integration platform to consolidate credit risk data across its operations in 12 African countries. This platform has enabled Barclays Africa to manage its credit risk more effectively and comply with the Basel III regulatory requirements.
ICBC (Industrial and Commercial Bank of China) has adopted advanced analytics to improve its credit risk management in China. Using machine learning algorithms, ICBC has analyzed vast amounts of data to identify patterns and predict future credit events. As a result, the bank has reportedly reduced its bad loans and improved its profitability.
These case studies highlight the transformative potential of data in credit risk management. As banks continue to harness the power of data, we can expect to see further advancements in credit risk management strategies and techniques.
The Advent of Advanced Analytics in Credit Risk Management
Understanding Advanced Analytics: The Basics
Advanced analytics is the sophisticated techniques used to predict future outcomes, trends, and behaviors based on historical data. It encompasses a range of methods, including predictive modeling, data mining, big data analytics, and machine learning. These techniques go beyond traditional analytics by providing deeper insights, more accurate predictions, and the ability to automate decision-making processes.
At the heart of advanced analytics is the ability to handle large and complex datasets. This includes structured data such as transaction records and unstructured data like social media posts or text documents. Advanced analytics algorithms can make sense of this data, uncover hidden patterns, and generate actionable insights.
How Advanced Analytics Transforms Credit Risk Management
Advanced analytics holds the potential to transform credit risk management fundamentally. For example, banks can develop more accurate and predictive credit risk models by applying sophisticated modeling techniques to large and diverse datasets.
For instance, a bank could use advanced analytics to identify correlations between a borrower’s credit risk and non-traditional factors such as their online shopping behavior or social media activity. This could enable the bank to assess better the risk of lending to customers who lack a traditional credit history.
Moreover, advanced analytics can enhance the speed and efficiency of credit risk management. For example, machine learning algorithms can automate assessing a loan application, reducing the time and resources required.
The Implementation of Advanced Analytics: Steps and Considerations
Implementing advanced analytics in credit risk management involves several key steps and considerations. First, it requires data infrastructure that can handle large volumes of diverse data. This may include investing in cloud storage solutions or big data platforms.
Next, banks need to develop or acquire the necessary analytics capabilities. This could involve hiring data scientists, partnering with analytics firms, or investing in training for existing staff.
Banks also need to consider the regulatory implications of using advanced analytics. For example, regulations such as the E.U.’s General Data Protection Regulation (GDPR) restrict how personal data can be used, which could impact specific analytics applications.
Finally, successfully implementing advanced analytics requires a culture that values data and analytics. This may involve fostering a mindset of data-driven decision-making and encouraging collaboration between data scientists and risk management professionals.
Case Studies: How Banks are Leveraging Advanced Analytics in Risk Management
Several banks have successfully leveraged advanced analytics in credit risk management.
One notable example is HSBC, which uses advanced analytics to predict the likelihood of default for individual borrowers. The bank uses machine learning algorithms to analyze a broad range of data, including economic trends, borrower characteristics, and loan details. This has reportedly helped HSBC to reduce its credit losses and improve its risk-adjusted return on capital.
Similarly, U.S. Bank uses advanced analytics to enhance its risk management. For example, the bank uses predictive models to forecast potential credit events and adjust its risk management strategies accordingly. As a result, U.S. Bank has reportedly improved its ability to manage credit risk and comply with regulatory requirements.
Commonwealth Bank has implemented an advanced analytics platform in Australia that consolidates risk data across its business lines. The platform uses machine learning algorithms to predict credit risk and provide actionable insights. This has enabled Commonwealth Bank to manage its risk more effectively and make informed lending decisions.
These case studies illustrate the transformative potential of advanced analytics in credit risk management. As banks continue to embrace this technology, we expect to see further improvements in credit risk management and overall banking operations.
The Role of Machine Learning and A.I. in Credit Risk Management
Machine Learning and A.I.: Revolutionizing the Banking Industry
Artificial Intelligence (A.I.) and Machine Learning (ML), subsets of advanced analytics, are revolutionizing the banking industry. A.I. refers to the simulation of human intelligence processes by machines. At the same time, ML is a type of A.I. that enables a system to learn from data rather than through explicit programming.
These technologies provide the ability to process and learn from vast amounts of data, leading to improved decision-making, increased efficiency, and the automation of numerous tasks. For example, A.I. and ML can enhance processes ranging from customer service and fraud detection to credit risk management in banking.
How Machine Learning and A.I. Can Enhance Credit Risk, Predictive Models
A.I. and ML can significantly enhance credit risk, predictive models. These technologies can analyze a vast array of structured and unstructured data and identify complex patterns and relationships that traditional methods might miss. For instance, ML models can evaluate the significance of various factors in a borrower’s ability to repay a loan and continuously improve their predictions based on new data.
ML can also automate much of the credit risk assessment process, making it faster and more efficient. For example, an A.I. system can quickly analyze a loan application and predict the likelihood of default, significantly speeding up the decision-making process.
Implementing Machine Learning and A.I.: Challenges and Solutions
Despite their potential, implementing A.I. and ML in credit risk management has several challenges. One is the need for high-quality, diverse data. If the data used to train an A.I. or ML model is biased or incomplete, this can lead to inaccurate predictions.
Another challenge is the ‘black box’ problem. ML models can be complex and opaque, making it difficult to understand how they make their predictions. This can be problematic from a regulatory perspective, as banks need to be able to explain their credit decisions.
One solution to these challenges is to use a hybrid approach, combining A.I. or ML with human expertise. While the A.I. or ML model can analyze vast amounts of data and identify patterns, human experts can provide context, interpret the model’s outputs, and ensure compliance with regulations.
Case Studies: Banks Winning with A.I. and Machine Learning in Risk Management
Numerous banks have successfully implemented A.I. and ML in credit risk management.
In the U.S., JP Morgan Chase uses machine learning to assess credit risk in its wholesale banking business. To predict a borrower’s default risk, the bank’s machine learning model evaluates various factors, including financial data, macroeconomic trends, and industry conditions.
In Europe, ING Bank uses A.I. to enhance its credit risk models. The bank’s A.I. system analyzes a broad range of data, including borrower characteristics, loan details, and economic indicators, to predict default risk. This has reportedly enabled ING Bank to improve its risk-adjusted return on capital.
In Asia, DBS Bank uses machine learning to predict credit card fraud, a related aspect of credit risk. The bank’s machine learning model analyzes transaction data to identify unusual patterns and alert the bank to potential fraud.
These case studies highlight the transformative potential of A.I. and ML in credit risk management. As more banks adopt these technologies, we expect to see more significant improvements in predicting and managing credit risk.
The Future of Credit Risk Management
Next-Generation Credit Risk Management: Predictions and Trends
The future of credit risk management is poised to be defined by increased digitization, further integration of advanced analytics, A.I., and machine learning, and a continued shift towards proactive risk management.
One prediction is that credit risk management will become even more data-driven. With the proliferation of data sources, especially from digital platforms and devices, banks will have more information to assess and manage credit risk. This will likely lead to the developing of more sophisticated predictive models that can provide real-time insights and early warning signals of potential credit events.
Another trend is the increasing use of A.I. and machine learning in credit risk management. These technologies will likely play an even more significant role in assessing credit risk, automating decision-making processes, providing personalized risk management solutions, and even predicting macroeconomic events that could impact credit risk.
The rise of FinTech and alternative lending platforms is also expected to shape the future of credit risk management. These platforms often use non-traditional data and innovative risk assessment methods, pushing traditional banks to innovate their credit risk management practices.
The Role of Regulatory Changes in Shaping the Future of Risk Management
Regulatory changes will continue to play a crucial role in shaping the future of credit risk management. For example, regulations such as the Basel III and IV accords impose stringent requirements on banks’ risk management practices, pushing them to improve their risk assessment capabilities and maintain adequate capital buffers.
Moreover, new regulations concerning data privacy and the use of A.I. could also impact credit risk management. For example, regulations restricting certain data types or requiring explainability in A.I. models could shape how banks use advanced analytics and A.I. in credit risk management.
While regulatory changes can pose challenges, they can also drive innovation in credit risk management. The need to comply with new regulations can push banks to seek new methods and technologies for managing credit risk.
Preparing for the Future: Skills and Mindset Shifts Needed for Risk Management Professionals
Preparing for the future of credit risk management requires both a shift in mindset and the development of new skills.
Regarding mindset, risk management professionals must embrace data and technology as crucial components of credit risk management. They need to move beyond seeing credit risk management as a compliance necessity and instead view it as a strategic function that can drive business performance and resilience.
In terms of skills, risk management professionals will need to become proficient in using advanced analytics and A.I. tools. This doesn’t mean that every risk manager needs to become a data scientist, but they will need to understand these tools and be able to work effectively with data professionals. In addition, data analysis, predictive modeling, and machine learning skills will become increasingly important.
Moreover, risk management professionals must develop their strategic thinking and decision-making skills. As credit risk management becomes more proactive and strategic, they will need to be able to interpret the outputs of predictive models, make informed decisions under uncertainty, and effectively communicate their risk assessments and decisions to stakeholders.
The future of credit risk management is undoubtedly complex. Still, with these shifts in mindset and skillsets, risk management professionals can turn challenges into opportunities and play a key role in shaping the future of banking.
Key Takeaways: Rethinking Credit Risk Management
Credit risk management is not a static, one-dimensional task. Instead, the ever-evolving economic landscape, emerging technologies, and changing regulatory requirements make it a dynamic and multifaceted responsibility. This e-book has underlined the need to rethink traditional methods and strategies of credit risk management.
Credit risk management has traditionally been seen as a compliance requirement, but it holds much greater potential. By shifting our mindset, we can see credit risk management as a strategic function that can enhance business resilience and performance. A well-managed credit portfolio can reduce losses, increase profitability, and enable a bank to respond more effectively to changes in the business environment.
Advanced analytics, machine learning, and A.I. offer powerful tools for transforming credit risk management. By harnessing these technologies, banks can better analyze and predict credit risk, improve decision-making speed and efficiency, and gain a competitive edge in the banking industry.
Final Thoughts on Embracing Advanced Technologies for Effective Risk Management
Embracing advanced technologies for credit risk management is not without its challenges. Banks must overcome data silos, invest in data infrastructure, and develop the necessary analytics capabilities. They must also manage the regulatory implications of using advanced analytics and A.I. and foster a culture that values data and analytics.
However, the benefits far outweigh the challenges. Case studies from leading banks like HSBC, U.S. Bank, Commonwealth Bank, JP Morgan Chase, ING Bank, and DBS Bank illustrate the transformative potential of these technologies. By applying advanced analytics and A.I., these banks have reduced credit losses, improved their risk-adjusted return on capital, and enhanced their risk management strategies.
As we move into the future, it’s clear that advanced analytics, A.I., and machine learning will play an increasingly significant role in credit risk management. Banks that can effectively harness these technologies will be well-placed to manage credit risk proactively, enhance their business performance, and navigate the ever-changing banking landscape.
Rethinking credit risk management and embracing advanced technologies is not just an option – it’s necessary for banks that want to thrive in the digital age. Let’s remember that with change comes opportunity. So let’s seize the opportunities these technologies offer and redefine the future of credit risk management together.