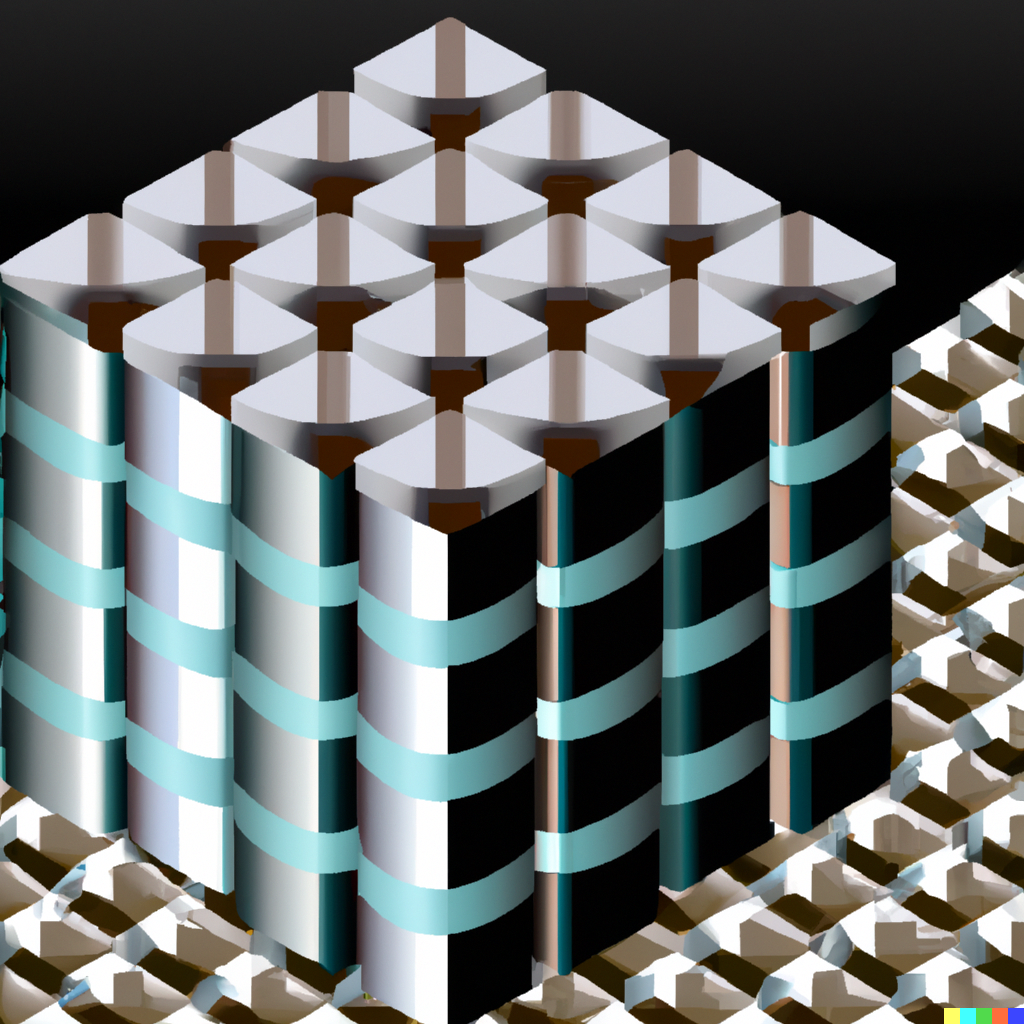
Enterprise Data Transformation in Financial Services
The ultimate guide to Enterprise Data Transformation in Financial Services, including data strategy, architecture, transformation roadmap, and implementation plan.
In today’s digital age, data has undeniably emerged as one of the most significant assets for any organization. But for financial enterprises, its value is paramount.
Enterprise Data Transformation in Financial Services: The Role and Value
Financial enterprises, whether they be banks, insurance companies, or investment firms, are navigating a sea of numbers daily. These numbers tell them everything – from a client’s transaction history and creditworthiness to market trends and investment opportunities. According to a study by McKinsey & Company, leading financial institutions that leverage data analytics have seen a profit increase of up to 20% and a 30% reduction in their provisioning costs.
Consider credit risk models, which rely on vast datasets to predict the likelihood of a borrower defaulting. Or, delve into algorithmic trading, where microseconds matter, and every bit of data can influence buy or sell decisions, affecting billions in assets. Simply put, data drives decision-making, risk management, customer service, and innovation in the financial world. It’s the foundation upon which successful strategies are built and executed.
Why Managing Data Remain a Challenge?
Despite its critical role, effectively managing data is no small feat for financial enterprises. The sheer volume of data is staggering. IDC reported that the global datasphere would grow to 175 zettabytes by 2025, with a significant chunk attributed to financial transactions and services.
Moreover, data in the financial sector is often siloed. Different departments, like credit, investment, and retail banking, might operate on separate platforms with limited interaction. This fragmentation can lead to inconsistencies, redundancies, and gaps in data. Additionally, the regulatory landscape is complex. Financial institutions face strict regulations like the General Data Protection Regulation (GDPR) in Europe or the Dodd-Frank Wall Street Reform in the U.S., which mandate specific data handling and reporting practices.
Furthermore, as cyber threats grow in sophistication, ensuring data security becomes an ever-increasing challenge. The cost of a data breach for financial enterprises isn’t just monetary; it can severely damage trust and reputation, taking years to rebuild.
The Strategic Imperative for Enterprise Data Transformation in Financial Services
Given these challenges, the need for a comprehensive data transformation strategy in financial services is not just preferable; it’s imperative. The potential rewards of harnessing data are vast: personalized client services, improved operational efficiencies, innovative financial products, and a more profound market understanding. Conversely, the risks of not addressing data challenges head-on are significant, from missed market opportunities to regulatory penalties.
But there’s a larger trend at play beyond the risks and rewards. Financial services are on the brink of a broader digital transformation characterized by artificial intelligence, blockchain technologies, and fintech collaborations. To navigate this transformation effectively and be competitive, having a robust, agile, and secure data strategy is not optional—it’s foundational.
As the financial landscape becomes increasingly digital and interconnected, data stands at the heart of opportunities and challenges. Financial enterprises that recognize the strategic imperative of data transformation and act on it will be the ones leading the charge into the future, while others risk falling behind.
Enterprise Data Transformation in Financial Services: Data Strategy
The Competitive Landscape
In the fast-paced world of financial services, having a cutting-edge can mean the difference between leading the market and struggling to keep pace. A report by the World Economic Forum highlighted that over 50% of global banking customers now use fintech products, emphasizing the increasing competition traditional banks face from agile, data-driven startups. These fintech firms capitalize on robust data strategies to quickly analyze market trends, understand consumer behaviors, and make informed decisions, often at a speed that larger, traditional institutions find challenging to match. Thus, for banks and financial organizations to retain their market share and attract new customers, a comprehensive data strategy isn’t just a nice-to-have—it’s a necessity.
Risk Management and Compliance
Financial institutions handle vast sums of money and sensitive information daily, making them prime targets for cyber-attacks. According to a study by Accenture, the financial sector faces cyber-attacks 300 times more frequently than other industries. An effective data strategy encompasses robust security protocols to fend off these threats.
Moreover, regulatory bodies across the globe have established stringent compliance requirements for financial entities. Whether it’s the European Union’s GDPR or the U.S.’s Sarbanes-Oxley Act, institutions are under pressure to ensure data integrity, accuracy, and security. A sound data strategy can help streamline compliance by ensuring that data handling, storage, and processing adhere to the ever-evolving regulatory landscape.
Enhancing Customer Experience
Today’s banking customers expect tailored experiences and instant responses, much like they receive from leading tech companies in other sectors. For instance, a study by Salesforce revealed that 75% of consumers expect consistent experiences across multiple channels (web, mobile, in-person, social). Leveraging data analytics can allow financial institutions to offer personalized product recommendations, anticipate customer needs, and resolve issues in real-time. By integrating data from various touchpoints, banks and financial firms can craft a seamless and personalized customer journey, turning satisfied customers into loyal advocates.
Innovation and New Product Development
The ability to harness and interpret data is a cornerstone for innovation in the financial sector. For example, insights derived from data analytics can reveal gaps in the market, prompting the development of new financial products tailored to niche customer segments. Additionally, financial institutions are exploring technologies like blockchain and artificial intelligence. A foundational data strategy is essential to maximize the benefits of these technologies. JPMorgan Chase’s development of its own cryptocurrency, JPM Coin, serves as an illustrative example. By utilizing blockchain technology and a data-centric approach, they innovated within the payment space, offering instantaneous transfers and settlements.
The significance of a data strategy in financial services cannot be understated. Data stands as the linchpin from maintaining a competitive edge and ensuring regulatory compliance to elevating customer experiences and fostering innovation. Financial institutions that recognize this and invest in building a robust data infrastructure will undoubtedly be better positioned to thrive in the modern financial landscape.
Enterprise Data Transformation in Financial Services: Building a Robust Data Strategy
Understanding Business Goals
The foundation of any effective data strategy lies in a deep understanding of its business goals. Before delving into data collection or analytics specifics, financial institutions must articulate what they aim to achieve. Is the goal to improve customer retention rates, streamline operational processes, or perhaps predict market trends more accurately? By 2020, Gartner had reported that over 80% of company leaders declared data as a critical part of their strategy. Still, only 10% felt they effectively used this data to drive business value. The discrepancy here underscores the importance of aligning data efforts with clear, tangible business objectives. Only when the end goals are clear can a strategy be devised to harness data effectively to serve them.
Data Identification and Categorization
Once the overarching goals have been established, institutions must identify the types of data that will help achieve those goals. This involves determining which datasets are already available and which new data sources might be needed.
For instance, if a bank wants to enhance customer experiences, it would be crucial to analyze transactional data, customer feedback, and online interaction data. Once identified, data must be categorized—often by type (e.g., transactional, behavioral, or qualitative), source (e.g., internal systems, third-party providers, or direct customer feedback), or relevance (primary vs. secondary data). This structured approach ensures that data is easily accessible and manageable when it’s needed.
Data Quality Management
The value of data is intrinsically linked to its quality. Poor data can lead to misguided strategies, misplaced investments, and lost opportunities. A study by IBM estimated that poor data quality costs the U.S. economy around $3.1 trillion a year. Therefore, financial institutions must implement rigorous data quality management protocols.
This involves processes for:
- Data validation: Ensuring data accuracy and consistency.
- Data cleansing: Identifying and correcting (or removing) errors and inconsistencies in data.
- Data enrichment: Augmenting existing datasets with additional, relevant information from external sources.
- Data auditing: Regularly checking and maintaining data quality over time.
Financial institutions can confidently derive insights and make data-driven decisions by ensuring high data quality.
Governance, Compliance, and Ethics
A robust data strategy is incomplete without considering governance, compliance, and ethics.
Data governance involves establishing clear ownership, stewardship, and accountability for data management. It defines who can access data, how it’s used, and the processes in place to ensure data quality and security.
Compliance, especially in the financial sector, is non-negotiable. With regulations like GDPR and the California Consumer Privacy Act (CCPA), institutions must ensure they collect, store, process, and share data in line with legal requirements.
Furthermore, the ethical use of data is gaining prominence. As A.I. and machine learning play bigger roles in data analysis, ensuring these technologies are free from biases and respecting customer privacy becomes paramount. A commitment to ethical data practices protects institutions from potential backlash and strengthens customer trust.
Constructing a robust data strategy is a meticulous endeavor that goes beyond merely collecting vast amounts of data. It requires alignment with business goals, methodical identification and categorization of data, stringent quality management, and a steadfast commitment to governance, compliance, and ethics. Financial institutions that master these components position themselves to unlock the full potential of their data, driving innovation and maintaining a competitive edge.
Enterprise Data Transformation in Financial Services: Overcoming Common Data Challenges
In the journey to harness the full potential of data, financial institutions invariably encounter a series of hurdles. Tackling these challenges, head-on ensures that data remains an asset rather than a liability.
Siloed Data Sources
Across many financial enterprises, data often resides in isolated systems, with different departments or teams maintaining separate databases. This fragmentation can lead to discrepancies, redundancies, and a lack of a single source of truth.
Solution: Adopting an integrated data platform can help consolidate and centralize data from diverse sources. Data integration tools and middleware technologies like Apache Kafka or Informatica can connect these disparate data sources, enabling a cohesive view. Moreover, fostering a culture of cross-departmental collaboration ensures a more unified approach to data management.
Legacy Systems and Infrastructures
Many financial institutions still rely on older systems that weren’t designed for today’s data-intensive operations. These legacy systems can be inflexible, inefficient, and often incompatible with modern data tools.
Solution: Migrating to cloud-based solutions or adopting a hybrid model can provide more agility and compatibility. While the upfront cost and effort of transitioning from legacy systems can be significant, the long-term benefits in terms of flexibility, efficiency, and integration capabilities justify the investment. An example is the Bank of New York Mellon Corp, which embarked on a multi-year initiative to modernize its legacy systems, resulting in increased operational efficiency and improved customer experiences.
Data Security Concerns
Given the sensitive nature of financial data, security breaches can have devastating consequences in terms of financial loss and reputational damage. According to a report by the Ponemon Institute, the average cost of a data breach in the financial sector stands at $5.86 million.
Solution: Implementing a multi-layered security strategy is paramount. This includes encryption, regular vulnerability assessments, intrusion detection systems, and robust access controls. Employee training is also crucial, as human error often plays a role in security breaches. Adopting frameworks like the Zero Trust Model, which operates on the principle of “never trust, always verify,” can further bolster data security measures.
Scalability and Performance
As financial institutions grow and diversify, the amount of data they handle also expands. Systems must store this increasing volume and process it efficiently to derive timely insights.
Solution: Leveraging scalable architectures, such as those provided by cloud solutions, allows organizations to adapt to fluctuating data demands. Distributed processing frameworks like Apache, Hadoop, and Spark ensure that large datasets are processed efficiently. Financial giants like Visa have successfully implemented scalable solutions, with its VisaNet system handling more than 65,000 transaction messages every second during peak times.
While the road to optimal data management in financial services is fraught with challenges, they are not insurmountable. By recognizing these challenges and strategically implementing solutions, institutions can ensure that their data infrastructures are robust, secure, and poised for the future.
Enterprise Data Transformation in Financial Services: Building a Roadmap
Embarking on a data transformation journey in the financial sector necessitates a structured and methodical approach. By following a systematic roadmap, institutions can ensure that they move from their current state to a desired future state in a coherent and efficient manner.
Assessing Current Data Capabilities
Starting Point: Before initiating any transformation, it’s vital to understand where the organization currently stands. This involves thoroughly assessing existing data infrastructure, processes, tools, and skills.
Steps:
- Audit Existing Systems: Identify the data systems in use, their capacities, limitations, and integration capabilities.
- Skill Assessment: Evaluate the expertise level of your team. Do they have the necessary skills to handle advanced data tools and techniques?
- Data Quality Review: Analyze the quality, consistency, and accuracy of the data currently in the system.
- Process Examination: Understand the current data lifecycle – from collection, storage, and analysis to usage.
For instance, according to Experian’s Global Data Management Research, only 51% of organizations globally believe their data is accurate. This insight underscores the need for a comprehensive initial assessment.
Defining the Vision and Setting Targets
Destination: Know where you’re heading. The vision should encapsulate what the organization aims to achieve through its data transformation initiative.
Steps:
- Goal Setting: Based on business objectives, define clear, measurable targets. This could include improving data accuracy rates by a certain percentage or reducing data processing times.
- Stakeholder Alignment: Engage key stakeholders, from C-suite executives to data analysts, ensuring everyone understands and buys into the vision.
- Blueprint Creation: Draft a visual representation or blueprint of the desired end-state of the data infrastructure.
Prioritization: Quick Wins vs. Long-term Projects
Path Selection: Not all transformation projects are created equal. Some may offer immediate benefits, while others lay the groundwork for future gains.
Steps:
- Identify Quick Wins: These projects can be executed swiftly and offer immediate ROI. For instance, implementing a new data visualization tool might provide instant insights for decision-makers.
- Chart Out Long-term Initiatives: These projects may take more time and investment but are crucial for the organization’s long-term data strategy. An example could be migrating from legacy systems to a cloud-based infrastructure.
- Balance Resources: Allocate resources judiciously, ensuring that while quick wins maintain momentum, long-term projects continue to receive the attention they deserve.
Technology Evaluation: Picking the Right Tools
Tools for the Journey: A transformation is only as effective as the tools used. Given the plethora of technology options available, making informed choices is critical.
Steps:
- Needs Analysis: Based on the defined vision and targets, list the organization’s technological needs.
- Market Research: Review available tools and solutions in the market. Look at industry benchmarks and case studies.
- Pilot Testing: Before full-scale implementation, run pilot tests on shortlisted technologies. This allows for a real-world assessment of their capabilities and compatibility.
- Training: Ensure staff receive adequate training on new tools to maximize utility and adoption.
The enterprise data transformation in financial services combines introspection, vision, prioritization, and astute technological choices. Organizations can navigate this journey smoothly by adhering to a well-defined roadmap, ensuring that data becomes a genuine strategic asset driving enterprise performance.
Key Elements of a Successful Data Transformation
Several core elements can significantly influence the financial sector’s data transformation trajectory. When adequately addressed, these components ensure the transformation’s success and pave the way for a sustainable data-centric future for the organization.
Data Culture: Embedding a Data-driven Mindset
The journey toward true data transformation starts with a cultural shift. The data itself, no matter how well organized or analyzed, offers limited value unless the broader organization embraces it.
Steps and Considerations:
- Top-down Advocacy: Leadership must champion this cultural change, emphasizing the value of data-driven decision-making.
- Employee Training: Beyond mere tool usage, training should instill an appreciation for data, emphasizing its role in shaping company outcomes.
- Open Forums: Regular sessions where teams share data-driven insights and success stories can instill a sense of collective pride and ownership.
For instance, Capital One, a financial giant, has been lauded for embedding a data-driven culture, from executive decisions to customer interactions.
Talent: Building and Upskilling the Right Team
No transformation succeeds without the right people steering it. The evolving data landscape demands a mix of traditional skills and contemporary expertise.
Steps and Considerations:
- Hiring Specialists: Bring in data scientists, analysts, and experts who can guide the organization’s data initiatives.
- Ongoing Training: The world of data and analytics is constantly evolving. Regular upskilling sessions ensure the team remains at the forefront of industry developments.
- Collaborative Approach: Encourage collaboration between data teams and other departments, fostering a mutual exchange of knowledge and insights.
Partnerships: Collaborating with Fintechs and Tech Vendors
The financial sector doesn’t have to navigate the data transformation journey alone. Collaborations can significantly amplify their efforts.
Steps and Considerations:
- Identify Gaps: Determine areas where external expertise could be beneficial, such as advanced analytics, A.I. modeling, or cybersecurity.
- Engage with Fintechs: Many fintech firms offer niche solutions tailored to the financial sector’s challenges. Engaging with them can provide fresh perspectives and innovative solutions.
- Tech Vendor Partnerships: Forming alliances with tech vendors, especially those specializing in data tools and platforms, can ensure the organization stays equipped with the best resources.
An illustrative example is J.P. Morgan’s partnership with fintech startups through its “In-Residence” program, which aims to discover and develop innovative financial solutions.
Continuous Improvement and Iteration
The realm of data is dynamic. A successful transformation acknowledges that the journey doesn’t have a finite end but requires consistent evolution.
Steps and Considerations:
- Feedback Loops: Implement systems to constantly gather feedback on data initiatives from stakeholders, both internal and external.
- Performance Metrics: Establish clear metrics to gauge the success of data projects, ensuring that they align with broader business objectives.
- Regular Reviews: Hold periodic reviews to assess the efficacy of implemented data strategies and tools, making adjustments as needed.
A successful data transformation in the financial sector is multi-faceted. It’s about cultivating the right mindset, marshaling the best talent, forging beneficial partnerships, and committing to an ongoing journey of improvement. Organizations that recognize and act upon these elements position themselves to reap the myriad benefits of a truly data-driven enterprise.
Enterprise Data Transformation in Financial Services: Implementation Plan
The actualization of a data transformation vision demands a systematic, phased approach. This segmented implementation ensures that the transformation remains manageable, yields tangible results at every step, and builds momentum for subsequent phases. Let’s delineate a five-phase plan:
Phase 1: Foundation – Infrastructure and Data Lakes
Objective: Establish a robust, scalable foundation to capture, store, and manage vast quantities of data.
Steps and Considerations:
- Infrastructure Setup: Prioritize cloud-based or hybrid infrastructure, leveraging their flexibility and scalability.
- Data Lake Creation: Establish data lakes—a centralized repository—to store structured and unstructured data from diverse sources. For instance, Goldman Sachs leverages data lakes to ensure accessibility and agility.
- Data Governance: Initiate best practices to ensure data integrity, security, and privacy right from the onset.
Phase 2: Data Integration – Connecting Siloed Systems
The objective is to bridge the gaps between fragmented data sources to achieve a unified, cohesive view of enterprise data.
Steps and Considerations:
- Middleware Deployment: Use middleware solutions like Apache Kafka to facilitate real-time data flows between disparate systems.
- Data Cleaning: Identify redundancies, inconsistencies, and inaccuracies in the data, rectifying them for a harmonized dataset.
- Master Data Management (MDM): Implement MDM practices to ensure the enterprise relies on a single version of the truth.
Phase 3: Analytics and Insights – Tools and Capabilities
Objective: Extract actionable insights from the amassed data.
Steps and Considerations:
- Tool Selection: Invest in business intelligence and analytical tools like Tableau or Power B.I. to transform raw data into comprehensible insights.
- Skill Development: Provide training to stakeholders, equipping them to utilize these tools effectively.
- Dashboard Creation: Design dashboards to represent key metrics, ensuring decision-makers can quickly access pivotal data insights.
Phase 4: Optimization – Real-time Analytics and Advanced ML
Objective: Elevate the analytical prowess to harness real-time insights and predictive capabilities.
Steps and Considerations:
- Streaming Analytics: Implement tools like Apache Flink or Spark Streaming for real-time data processing and analytics.
- Machine Learning Models: Deploy machine learning to predict trends, detect anomalies, and enhance decision-making. Banks like Wells Fargo use ML for fraud detection, credit risk modeling, and customer service improvements.
- Automated Decisioning: Integrate ML insights into business processes to enable automated, data-driven decisions.
Phase 5: Monetization and Innovation – Creating New Revenue Streams
Objective: Use data insights for internal improvements and as a catalyst for revenue generation and innovation.
Steps and Considerations:
- Product Development: Leverage insights to develop or refine financial products tailored to customer needs and market demands.
- Data-as-a-Service (DaaS): Consider offering cleaned and processed data sets to external parties, establishing a new revenue stream. Companies like Experian, traditionally a credit reporting agency, have expanded into DaaS, providing business data insights.
- Innovation Labs: Create labs or incubators to constantly experiment with data-driven solutions, fostering a culture of continuous innovation.
A well-structured implementation plan, sequenced from foundational work to monetization, ensures that the data transformation journey remains strategic, focused, and outcome-oriented. Financial enterprises that proceed with such meticulous planning stand to optimize their current operations and uncover new avenues of growth and innovation.
Enterprise Data Transformation in Financial Services: Best Practices and Case Studies
The data transformation journey in the financial sector has seen both soaring successes and instructive missteps. By examining these, organizations can extract best practices, derive inspiration, and circumvent potential pitfalls.
Success Stories from Leading Financial Enterprises
- JPMorgan Chase & Co. – A.I. in Risk Management: JPMorgan adopted A.I. to assess risk and make lending decisions. By analyzing vast quantities of data, including non-traditional sources like social media, they’ve improved the accuracy of their risk models, resulting in better lending decisions and reduced defaults.
- Bank of America – Erica, the Virtual Financial Assistant: Powered by data analytics and cognitive messaging, Erica assists millions of clients with their banking needs, offering personalized financial advice, which has led to enhanced customer satisfaction and operational efficiency.
- HSBC – Fighting Fraud with Data: HSBC employed big data analytics to detect fraudulent activities in real time. The bank decreased fraudulent transactions by analyzing transaction patterns and leveraging machine learning, saving millions annually.
Lessons Learned: What to Do and What to Avoid
Do:
- Prioritize Data Security: Given the sensitivity of financial data, prioritize encryption, regular audits, and multi-factor authentication.
- Invest in Training: Ensure that technical teams and end-users receive training to maximize the data tools and platforms.
- Engage Stakeholders: Involve all stakeholders in the data transformation journey, from top executives to frontline employees.
Avoid:
- Data Hoarding: Not all data is useful. Collecting excessive data can clutter systems and dilute insights. Focus on quality over quantity.
- Underestimating Change Resistance: Employees accustomed to traditional methods might resist new, data-driven approaches. Address this with proper change management strategies.
- Over-reliance on Technology: While tools and platforms are essential, human judgment remains crucial. Balance technological insights with human expertise.
Innovative Applications of Data in Finance
- Personalized Banking with A.I.: Banks like Capital One employ A.I. to analyze customers’ spending habits, credit history, and personal preferences to offer tailor-made financial advice and product recommendations.
- Blockchain for Transparent Transactions: Several financial enterprises leverage blockchain, a data-driven technology, to create transparent, immutable, and efficient transaction systems. For instance, Santander launched the world’s first blockchain-based bond, ushering in a new era of transparent securities trading.
- Predictive Analysis for Stock Trading: Investment firms are increasingly using data analytics to predict stock market movements. Renaissance Technologies, one of the world’s most successful hedge funds, relies heavily on quantitative models and data analytics, yielding consistently impressive returns.
The landscape of the financial sector is dotted with compelling narratives of data-driven transformations. These stories serve as beacons, guiding enterprises toward best practices, innovative applications, and lessons learned. As the sector continues to evolve, these tales of innovation and insight will undoubtedly multiply, offering richer learnings for future aspirants.
Enterprise Data Transformation in Financial Services: Measuring the Success of Your Data Strategy
In the rapidly evolving world of financial data, merely implementing a strategy isn’t enough; measuring its efficacy is vital. A rigorous evaluative approach ensures that the data strategy remains aligned with organizational goals, delivers tangible benefits, and evolves as per the changing landscape.
Key Performance Indicators (KPIs)
KPIs provide quantifiable measurements to gauge the performance of your data strategy against predetermined objectives.
- Data Quality Score: This evaluates data’s accuracy, consistency, and freshness. High scores indicate trustworthy data that can underpin critical decisions.
- Time-to-Insight: Measure the time taken from data collection to derive actionable insights. A shorter span typically suggests efficiency and agility.
- User Engagement Metrics: Track the frequency and manner in which end-users interact with data tools, platforms, and dashboards. Higher engagement often points to the strategy’s relevance and effectiveness.
- Data Storage and Retrieval Efficiency: Monitor the speed and reliability with which data can be stored and retrieved from databases or data lakes.
For instance, Citigroup uses a range of KPIs to monitor its data initiatives, ensuring that they remain agile and relevant to the bank’s evolving needs.
Return on Investment (ROI) Calculations
ROI offers a clear lens into the financial effectiveness of your data strategy, revealing whether the benefits outweigh the costs.
- Direct Financial Gains: Calculate the revenue generated or costs saved directly from data-driven initiatives, such as personalized marketing campaigns or efficient risk management.
- Operational Efficiency Savings: Determine cost savings from improved operations, such as reduced data redundancy or faster data retrieval times.
- Long-term ROI: Include the valuation of intangible assets, such as improved customer satisfaction, enhanced brand reputation, or a more data-literate workforce.
For example, Wells Fargo implemented data analytics in its mortgage division and found that for every dollar invested in the initiative, they observed a return of almost four dollars due to more efficient lending decisions and reduced defaults.
Feedback Loops and Continuous Learning
Constructive feedback mechanisms ensure that the strategy remains dynamic and responsive to changing needs and challenges.
- User Feedback Surveys: Regularly solicit feedback from data tool and platform users, gathering insights about user-friendliness, utility, and areas of improvement.
- Data Strategy Audits: Conduct periodic audits to evaluate the alignment of the data strategy with broader business goals. This can uncover gaps or redundancies.
- External Benchmarking: Compare your organization’s data performance metrics against industry standards or leading competitors to identify strengths and areas of improvement.
Morgan Stanley, for instance, has established robust feedback mechanisms for its data initiatives. These feedback loops played a pivotal role when they shifted to a more integrated data infrastructure, as they could quickly adjust based on real-time user insights and industry benchmarks.
As with any strategic initiative, the success of a data strategy in the financial sector should be consistently measured and scrutinized. Key Performance Indicators, ROI calculations, and feedback loops serve as navigational beacons, ensuring the strategy delivers value, remains agile, and propels the enterprise toward its envisioned data-driven future.
Future of Data in Financial Services
The future of financial services is inexorably linked with the continued evolution of data. As technologies progress, the ways in which financial institutions harness, interpret, and leverage data will determine their ability to innovate, satisfy customers, and maintain a competitive edge. Here’s a glance into the future of data in the financial realm:
Predictive and Prescriptive Analytics
Financial institutions are progressing beyond the realm of descriptive analytics, which merely paints a picture of past events, and are rapidly embracing forward-looking analytics.
- Predictive Analytics: By leveraging machine learning and statistical algorithms, predictive analytics can forecast future events based on historical data. For example, credit card companies like Mastercard can predict the likelihood of a transaction being fraudulent and take proactive preventive measures.
- Prescriptive Analytics: Going a step further, prescriptive analytics provides recommendations on handling a future scenario. In wealth management, firms can offer real-time investment advice to clients based on market predictions, ensuring optimal portfolio performance.
Integration with A.I. and Automation
The symbiosis of A.I., automation, and data promises to reshape the contours of financial services.
- AI-Powered Financial Advisors: Robo-advisors, which rely on A.I. algorithms to provide investment advice without human intervention, have seen burgeoning popularity. With its Digital Advisor service, Vanguard is capitalizing on this trend, offering tailored investment strategies based on individual financial goals and risk tolerance.
- Automated Regulatory Compliance: With regulations in the financial sector becoming increasingly intricate, A.I. can help banks automatically stay compliant by parsing through voluminous regulatory texts and aligning bank operations accordingly.
- Real-time Customer Service: Integrating A.I. with customer data allows chatbots and virtual assistants to offer personalized, instant responses to client queries, significantly enhancing user experience.
Regulatory and Ethical Considerations
As data’s role magnifies, so do the responsibilities of financial institutions to handle it judiciously.
- Data Privacy Laws: Financial institutions must ensure stringent data privacy measures with regulations like the General Data Protection Regulation (GDPR) in Europe and the California Consumer Privacy Act (CCPA) in the U.S.. Non-compliance can result in hefty penalties.
- Ethical Use of A.I. and Data: Just because A.I. can decide based on data doesn’t always mean it should. To prevent algorithmic biases, financial institutions need to strike a balance between AI-driven automation and human judgment, especially in sensitive areas like loan approvals.
- Transparency in Data Usage: As customers become more data-savvy, they demand transparency in how their data is used. Banks and financial firms must be forthright about their data practices to maintain trust.
The future of data in financial services is poised to be exciting, transformative, and, inevitably, challenging. The seamless integration of predictive analytics, A.I., and automation will offer unprecedented capabilities. Yet, with great power comes great responsibility. As financial institutions stand on the cusp of this data-driven future, they must tread with a blend of ambition and caution, ensuring that innovation never comes at the cost of ethics or customer trust.
The kaleidoscope of financial services is in a perpetual state of flux, largely driven by the dynamism of data. As we look back at the nuances of data transformation, a few overarching themes emerge, guiding financial enterprises into the future.
The Continuous Journey of Data Transformation
Data transformation isn’t a one-time project but an ongoing journey. As the financial landscape evolves, so too do enterprises’ data needs and strategies.
- Adaptability: Just as the New York Stock Exchange adapted to algorithmic trading, replacing the clamor of traders with the hum of machines, financial enterprises must be nimble, ready to pivot their data strategies in response to industry shifts.
- Ongoing Education: Today’s tools, platforms, and methodologies might be obsolete tomorrow. Constant upskilling and training become paramount. Goldman Sachs, for instance, has been proactive in training its employees in Python, preparing them for a more data-centric environment.
Preparing for Future Disruptions and Opportunities
The horizon of financial services is dotted with both challenges and prospects, and data stands at the fulcrum.
- Proactive Approach: It’s crucial for financial institutions to not just react to disruptions but to anticipate them. With robust data analytics, for instance, banks can forecast emerging market trends, from cryptocurrency evolutions to shifts in consumer spending habits.
- Collaboration: In an interconnected digital world, silos are detrimental. Partnerships with fintechs, tech vendors, and even competitors can unlock synergistic value. JPMorgan Chase’s collaboration with OnDeck to streamline and enhance small business lending is a testament to such alliances’ power.
Recommended Data Management Tools and Platforms for Financial Enterprises
Selecting the right tools is pivotal in harnessing the full potential of data.
- Snowflake: This cloud data platform has seen rapid adoption for allowing multiple teams to work on data simultaneously without compromising performance.
- Tableau: Tableau stands out for data visualization and business intelligence, enabling financial analysts to derive actionable insights from complex datasets.
- Talend: As a robust data integration tool, Talend assists financial enterprises in collating data from disparate sources, ensuring cohesion and accuracy.
- IBM Guardium: Given the critical importance of data security in finance, Guardium provides real-time activity monitoring and vulnerability assessment, ensuring that sensitive financial data remains uncompromised.
- Alteryx: This end-to-end platform offers data blending and advanced analytics and is particularly favored for its user-friendly interface, empowering even non-technical users.
In the annals of financial history, the present era will be remembered for its data renaissance. As we stand on this precipice, looking into the future, the promise of what data can achieve is boundless. Armed with the right strategies, tools, and ethos, financial enterprises can not only navigate this complex terrain but also redefine it, charting a course for unprecedented growth and innovation.